Same stochastic momenta => identical distribution
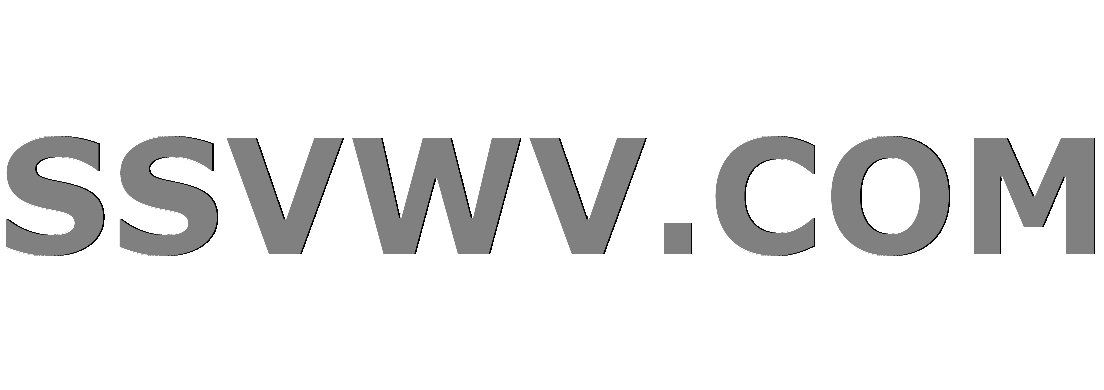
Multi tool use
I got a stochastic problem and hope some of you can help me!
It's about stochastic variables $X,Y in L^{infty}(P)$, which have the same (finite) stochstic moments $forall n in mathbb{N}: ~mathbb{E}X^n = mathbb{E}Y^n $
(a) First I need to show, that for an arbitrary integrable and continous function $f $ follows: $mathbb{E}f(X) = mathbb{E}f(Y)$
(b) Furthermore I should show, that the distributions $X$ and $Y$ even are identical.
I've already thought about the two problems.
I guess the integrability is only necessary for definig/calculation $mathbb{E}f(X)$, so this is not the propoerty, which is used in the proof. My approach for (a) was the Weierstraß - Theorem. The theorem claims, that $forall f in C([a,b])$ with $-infty < a < b < infty$ there holds: $forall epsilon >0 ~ exists n in mathbb{N}: exists p in P_n: ||f-p ||_{infty} < epsilon$ on $[a,b]$.
So for a continous $f$ I can approximate it by $p in P_n$: $f(z) approx sum_{k=0}^{n} a_k z^k$. So it follows $mathbb{E}f(X) = mathbb{E}left[ sum_{k=0}^{n} a_k z^k right] = sum_{k=0}^{n} a_k mathbb{E}X^n = sum_{k=0}^{n} a_k mathbb{E}Y^n = mathbb{E}f(Y)$.
At least that's the plan. The requirement for the Weierstraß-Theorem was a finite interval $[a,b]$, but according to the indication I cannot restrain to finite $a,b$. How can I generalize my result? (Or is there a general misstake in my approach?)
For (b) I dont't have an idea how to proof this. It might be possible, that I Need to apply a) but don't see how, due to I made a misstake at a)?
I am a little bit confused, due to I thought $X,Y$ having same stochastical momenta is equal to $X$ idential to $Y$. But obviously this is exactly what we need to proof.
I hope some of you can me!
Thanks a lot! :)
probability-theory measure-theory probability-distributions
add a comment |
I got a stochastic problem and hope some of you can help me!
It's about stochastic variables $X,Y in L^{infty}(P)$, which have the same (finite) stochstic moments $forall n in mathbb{N}: ~mathbb{E}X^n = mathbb{E}Y^n $
(a) First I need to show, that for an arbitrary integrable and continous function $f $ follows: $mathbb{E}f(X) = mathbb{E}f(Y)$
(b) Furthermore I should show, that the distributions $X$ and $Y$ even are identical.
I've already thought about the two problems.
I guess the integrability is only necessary for definig/calculation $mathbb{E}f(X)$, so this is not the propoerty, which is used in the proof. My approach for (a) was the Weierstraß - Theorem. The theorem claims, that $forall f in C([a,b])$ with $-infty < a < b < infty$ there holds: $forall epsilon >0 ~ exists n in mathbb{N}: exists p in P_n: ||f-p ||_{infty} < epsilon$ on $[a,b]$.
So for a continous $f$ I can approximate it by $p in P_n$: $f(z) approx sum_{k=0}^{n} a_k z^k$. So it follows $mathbb{E}f(X) = mathbb{E}left[ sum_{k=0}^{n} a_k z^k right] = sum_{k=0}^{n} a_k mathbb{E}X^n = sum_{k=0}^{n} a_k mathbb{E}Y^n = mathbb{E}f(Y)$.
At least that's the plan. The requirement for the Weierstraß-Theorem was a finite interval $[a,b]$, but according to the indication I cannot restrain to finite $a,b$. How can I generalize my result? (Or is there a general misstake in my approach?)
For (b) I dont't have an idea how to proof this. It might be possible, that I Need to apply a) but don't see how, due to I made a misstake at a)?
I am a little bit confused, due to I thought $X,Y$ having same stochastical momenta is equal to $X$ idential to $Y$. But obviously this is exactly what we need to proof.
I hope some of you can me!
Thanks a lot! :)
probability-theory measure-theory probability-distributions
Your random variables are given to be bounded a.s., so you can take the relevant $[a,b]$ in the Weierstrass theorem to be $[-M,M]$ where $M$ is the $L^infty$ norm of the variables. Now $E[p(X)]=E[p(Y)]$ for any polynomial $p$, and now you just need to improve that to $E[f(X)]=E[f(Y)]$ for any bounded continuous function $f$.
– Ian
Dec 10 '18 at 21:21
@lan Thanks for your quick respond. That solves my problem with a)!
– pcalc
Dec 10 '18 at 21:40
Moments don't always determine the distribution, but do so in many cases. Maybe relevant: mathoverflow.net/questions/3525/…
– jdods
Dec 11 '18 at 1:23
@jdods But here the variables are given to be bounded a.s., which is well more than enough.
– Ian
Dec 11 '18 at 15:43
add a comment |
I got a stochastic problem and hope some of you can help me!
It's about stochastic variables $X,Y in L^{infty}(P)$, which have the same (finite) stochstic moments $forall n in mathbb{N}: ~mathbb{E}X^n = mathbb{E}Y^n $
(a) First I need to show, that for an arbitrary integrable and continous function $f $ follows: $mathbb{E}f(X) = mathbb{E}f(Y)$
(b) Furthermore I should show, that the distributions $X$ and $Y$ even are identical.
I've already thought about the two problems.
I guess the integrability is only necessary for definig/calculation $mathbb{E}f(X)$, so this is not the propoerty, which is used in the proof. My approach for (a) was the Weierstraß - Theorem. The theorem claims, that $forall f in C([a,b])$ with $-infty < a < b < infty$ there holds: $forall epsilon >0 ~ exists n in mathbb{N}: exists p in P_n: ||f-p ||_{infty} < epsilon$ on $[a,b]$.
So for a continous $f$ I can approximate it by $p in P_n$: $f(z) approx sum_{k=0}^{n} a_k z^k$. So it follows $mathbb{E}f(X) = mathbb{E}left[ sum_{k=0}^{n} a_k z^k right] = sum_{k=0}^{n} a_k mathbb{E}X^n = sum_{k=0}^{n} a_k mathbb{E}Y^n = mathbb{E}f(Y)$.
At least that's the plan. The requirement for the Weierstraß-Theorem was a finite interval $[a,b]$, but according to the indication I cannot restrain to finite $a,b$. How can I generalize my result? (Or is there a general misstake in my approach?)
For (b) I dont't have an idea how to proof this. It might be possible, that I Need to apply a) but don't see how, due to I made a misstake at a)?
I am a little bit confused, due to I thought $X,Y$ having same stochastical momenta is equal to $X$ idential to $Y$. But obviously this is exactly what we need to proof.
I hope some of you can me!
Thanks a lot! :)
probability-theory measure-theory probability-distributions
I got a stochastic problem and hope some of you can help me!
It's about stochastic variables $X,Y in L^{infty}(P)$, which have the same (finite) stochstic moments $forall n in mathbb{N}: ~mathbb{E}X^n = mathbb{E}Y^n $
(a) First I need to show, that for an arbitrary integrable and continous function $f $ follows: $mathbb{E}f(X) = mathbb{E}f(Y)$
(b) Furthermore I should show, that the distributions $X$ and $Y$ even are identical.
I've already thought about the two problems.
I guess the integrability is only necessary for definig/calculation $mathbb{E}f(X)$, so this is not the propoerty, which is used in the proof. My approach for (a) was the Weierstraß - Theorem. The theorem claims, that $forall f in C([a,b])$ with $-infty < a < b < infty$ there holds: $forall epsilon >0 ~ exists n in mathbb{N}: exists p in P_n: ||f-p ||_{infty} < epsilon$ on $[a,b]$.
So for a continous $f$ I can approximate it by $p in P_n$: $f(z) approx sum_{k=0}^{n} a_k z^k$. So it follows $mathbb{E}f(X) = mathbb{E}left[ sum_{k=0}^{n} a_k z^k right] = sum_{k=0}^{n} a_k mathbb{E}X^n = sum_{k=0}^{n} a_k mathbb{E}Y^n = mathbb{E}f(Y)$.
At least that's the plan. The requirement for the Weierstraß-Theorem was a finite interval $[a,b]$, but according to the indication I cannot restrain to finite $a,b$. How can I generalize my result? (Or is there a general misstake in my approach?)
For (b) I dont't have an idea how to proof this. It might be possible, that I Need to apply a) but don't see how, due to I made a misstake at a)?
I am a little bit confused, due to I thought $X,Y$ having same stochastical momenta is equal to $X$ idential to $Y$. But obviously this is exactly what we need to proof.
I hope some of you can me!
Thanks a lot! :)
probability-theory measure-theory probability-distributions
probability-theory measure-theory probability-distributions
asked Dec 10 '18 at 21:15
pcalc
27518
27518
Your random variables are given to be bounded a.s., so you can take the relevant $[a,b]$ in the Weierstrass theorem to be $[-M,M]$ where $M$ is the $L^infty$ norm of the variables. Now $E[p(X)]=E[p(Y)]$ for any polynomial $p$, and now you just need to improve that to $E[f(X)]=E[f(Y)]$ for any bounded continuous function $f$.
– Ian
Dec 10 '18 at 21:21
@lan Thanks for your quick respond. That solves my problem with a)!
– pcalc
Dec 10 '18 at 21:40
Moments don't always determine the distribution, but do so in many cases. Maybe relevant: mathoverflow.net/questions/3525/…
– jdods
Dec 11 '18 at 1:23
@jdods But here the variables are given to be bounded a.s., which is well more than enough.
– Ian
Dec 11 '18 at 15:43
add a comment |
Your random variables are given to be bounded a.s., so you can take the relevant $[a,b]$ in the Weierstrass theorem to be $[-M,M]$ where $M$ is the $L^infty$ norm of the variables. Now $E[p(X)]=E[p(Y)]$ for any polynomial $p$, and now you just need to improve that to $E[f(X)]=E[f(Y)]$ for any bounded continuous function $f$.
– Ian
Dec 10 '18 at 21:21
@lan Thanks for your quick respond. That solves my problem with a)!
– pcalc
Dec 10 '18 at 21:40
Moments don't always determine the distribution, but do so in many cases. Maybe relevant: mathoverflow.net/questions/3525/…
– jdods
Dec 11 '18 at 1:23
@jdods But here the variables are given to be bounded a.s., which is well more than enough.
– Ian
Dec 11 '18 at 15:43
Your random variables are given to be bounded a.s., so you can take the relevant $[a,b]$ in the Weierstrass theorem to be $[-M,M]$ where $M$ is the $L^infty$ norm of the variables. Now $E[p(X)]=E[p(Y)]$ for any polynomial $p$, and now you just need to improve that to $E[f(X)]=E[f(Y)]$ for any bounded continuous function $f$.
– Ian
Dec 10 '18 at 21:21
Your random variables are given to be bounded a.s., so you can take the relevant $[a,b]$ in the Weierstrass theorem to be $[-M,M]$ where $M$ is the $L^infty$ norm of the variables. Now $E[p(X)]=E[p(Y)]$ for any polynomial $p$, and now you just need to improve that to $E[f(X)]=E[f(Y)]$ for any bounded continuous function $f$.
– Ian
Dec 10 '18 at 21:21
@lan Thanks for your quick respond. That solves my problem with a)!
– pcalc
Dec 10 '18 at 21:40
@lan Thanks for your quick respond. That solves my problem with a)!
– pcalc
Dec 10 '18 at 21:40
Moments don't always determine the distribution, but do so in many cases. Maybe relevant: mathoverflow.net/questions/3525/…
– jdods
Dec 11 '18 at 1:23
Moments don't always determine the distribution, but do so in many cases. Maybe relevant: mathoverflow.net/questions/3525/…
– jdods
Dec 11 '18 at 1:23
@jdods But here the variables are given to be bounded a.s., which is well more than enough.
– Ian
Dec 11 '18 at 15:43
@jdods But here the variables are given to be bounded a.s., which is well more than enough.
– Ian
Dec 11 '18 at 15:43
add a comment |
0
active
oldest
votes
Your Answer
StackExchange.ifUsing("editor", function () {
return StackExchange.using("mathjaxEditing", function () {
StackExchange.MarkdownEditor.creationCallbacks.add(function (editor, postfix) {
StackExchange.mathjaxEditing.prepareWmdForMathJax(editor, postfix, [["$", "$"], ["\\(","\\)"]]);
});
});
}, "mathjax-editing");
StackExchange.ready(function() {
var channelOptions = {
tags: "".split(" "),
id: "69"
};
initTagRenderer("".split(" "), "".split(" "), channelOptions);
StackExchange.using("externalEditor", function() {
// Have to fire editor after snippets, if snippets enabled
if (StackExchange.settings.snippets.snippetsEnabled) {
StackExchange.using("snippets", function() {
createEditor();
});
}
else {
createEditor();
}
});
function createEditor() {
StackExchange.prepareEditor({
heartbeatType: 'answer',
autoActivateHeartbeat: false,
convertImagesToLinks: true,
noModals: true,
showLowRepImageUploadWarning: true,
reputationToPostImages: 10,
bindNavPrevention: true,
postfix: "",
imageUploader: {
brandingHtml: "Powered by u003ca class="icon-imgur-white" href="https://imgur.com/"u003eu003c/au003e",
contentPolicyHtml: "User contributions licensed under u003ca href="https://creativecommons.org/licenses/by-sa/3.0/"u003ecc by-sa 3.0 with attribution requiredu003c/au003e u003ca href="https://stackoverflow.com/legal/content-policy"u003e(content policy)u003c/au003e",
allowUrls: true
},
noCode: true, onDemand: true,
discardSelector: ".discard-answer"
,immediatelyShowMarkdownHelp:true
});
}
});
Sign up or log in
StackExchange.ready(function () {
StackExchange.helpers.onClickDraftSave('#login-link');
});
Sign up using Google
Sign up using Facebook
Sign up using Email and Password
Post as a guest
Required, but never shown
StackExchange.ready(
function () {
StackExchange.openid.initPostLogin('.new-post-login', 'https%3a%2f%2fmath.stackexchange.com%2fquestions%2f3034492%2fsame-stochastic-momenta-identical-distribution%23new-answer', 'question_page');
}
);
Post as a guest
Required, but never shown
0
active
oldest
votes
0
active
oldest
votes
active
oldest
votes
active
oldest
votes
Thanks for contributing an answer to Mathematics Stack Exchange!
- Please be sure to answer the question. Provide details and share your research!
But avoid …
- Asking for help, clarification, or responding to other answers.
- Making statements based on opinion; back them up with references or personal experience.
Use MathJax to format equations. MathJax reference.
To learn more, see our tips on writing great answers.
Some of your past answers have not been well-received, and you're in danger of being blocked from answering.
Please pay close attention to the following guidance:
- Please be sure to answer the question. Provide details and share your research!
But avoid …
- Asking for help, clarification, or responding to other answers.
- Making statements based on opinion; back them up with references or personal experience.
To learn more, see our tips on writing great answers.
Sign up or log in
StackExchange.ready(function () {
StackExchange.helpers.onClickDraftSave('#login-link');
});
Sign up using Google
Sign up using Facebook
Sign up using Email and Password
Post as a guest
Required, but never shown
StackExchange.ready(
function () {
StackExchange.openid.initPostLogin('.new-post-login', 'https%3a%2f%2fmath.stackexchange.com%2fquestions%2f3034492%2fsame-stochastic-momenta-identical-distribution%23new-answer', 'question_page');
}
);
Post as a guest
Required, but never shown
Sign up or log in
StackExchange.ready(function () {
StackExchange.helpers.onClickDraftSave('#login-link');
});
Sign up using Google
Sign up using Facebook
Sign up using Email and Password
Post as a guest
Required, but never shown
Sign up or log in
StackExchange.ready(function () {
StackExchange.helpers.onClickDraftSave('#login-link');
});
Sign up using Google
Sign up using Facebook
Sign up using Email and Password
Post as a guest
Required, but never shown
Sign up or log in
StackExchange.ready(function () {
StackExchange.helpers.onClickDraftSave('#login-link');
});
Sign up using Google
Sign up using Facebook
Sign up using Email and Password
Sign up using Google
Sign up using Facebook
Sign up using Email and Password
Post as a guest
Required, but never shown
Required, but never shown
Required, but never shown
Required, but never shown
Required, but never shown
Required, but never shown
Required, but never shown
Required, but never shown
Required, but never shown
fS3ddJNcwr KuvOJGZDaAiIui p8itosiw QK,6Ad28x TIOy vi5s4uQ
Your random variables are given to be bounded a.s., so you can take the relevant $[a,b]$ in the Weierstrass theorem to be $[-M,M]$ where $M$ is the $L^infty$ norm of the variables. Now $E[p(X)]=E[p(Y)]$ for any polynomial $p$, and now you just need to improve that to $E[f(X)]=E[f(Y)]$ for any bounded continuous function $f$.
– Ian
Dec 10 '18 at 21:21
@lan Thanks for your quick respond. That solves my problem with a)!
– pcalc
Dec 10 '18 at 21:40
Moments don't always determine the distribution, but do so in many cases. Maybe relevant: mathoverflow.net/questions/3525/…
– jdods
Dec 11 '18 at 1:23
@jdods But here the variables are given to be bounded a.s., which is well more than enough.
– Ian
Dec 11 '18 at 15:43