Identically distributed vs P(X > Y) = P(Y > X)
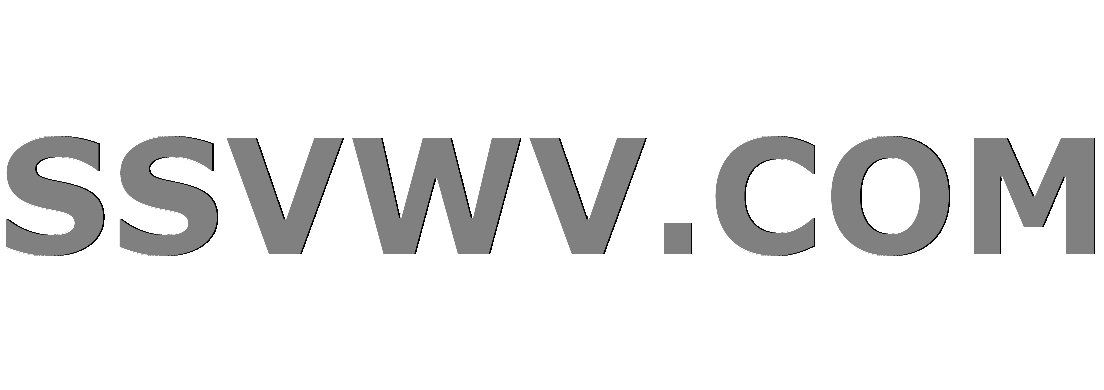
Multi tool use
$begingroup$
I've two related propositions which seem correct intuitively, but I struggle to prove them properly.
Question 1
Prove or disprove: If $X$ and $Y$ are independent and have identical marginal distributions, then $mathbb{P} (Y > X) = mathbb{P} (X > Y) = 1/2$
Due to independence, the joint PDF of $X$ and $Y$ is the product of their marginal PDF:
$$ begin{align}
mathbb{P} (Y > X) &= int_{-infty}^infty int_x^infty p(x) , p(y) , dy , dx \
mathbb{P} (X > Y) &= int_{-infty}^infty int_y^infty p(x) , p(y) , dx , dy
= int_{-infty}^infty int_x^infty p(y) , p(x) , dy , dx
end{align} $$
The last step is based on the fact that the integral won't change if we simply rename the integration parameters $x$ and $y$ consistently. So we have shown that $mathbb{P} (Y > X) = mathbb{P} (X > Y)$
Side note: Even if $X$ and $Y$ are dependent, this result still holds so long as their joint PDF is exchangeable i.e. $p(x, y) = p(y, x)$
Let $u = y - x$ so that
$$ mathbb{P} (Y > X) = int_{-infty}^infty int_0^infty p(x) , p(u + x) , du , dx $$
I thought of applying Fubini's theorem but it doesn't help to show that it's equal to 1/2, so maybe it's not 1/2?
Alternatively, consider that
$$ mathbb{P} (Y > X) + mathbb{P} (X > Y) + mathbb{P} (Y = X) = 1 $$
If we assume that $mathbb{P} (Y = X) = 0$ then we can conclude that $mathbb{P} (Y > X) = 1/2$. But is this assumption justified?
Question 2
Prove or disprove: If $X$ and $Y$ are independent and $mathbb{P} (Y > X) = mathbb{P} (X > Y)$, then they have identical marginal distributions. If this statement is true, then is it still true if $X$ and $Y$ are dependent?
@Xi'an provided a counter-example. Suppose that
$$ begin{bmatrix} X \ Y end{bmatrix}
sim mathcal{N} left(
begin{bmatrix} mu \ mu end{bmatrix},
begin{bmatrix} sigma_1^2 & c \ c & sigma_2^2 end{bmatrix}
right)$$
Then $X-Y$ and $Y-X$ have the same distribution: $mathcal{N} left(0, sigma_1^2 + sigma_2^2 - 2c right)$ and hence $mathbb{P} (Y - X > 0) = mathbb{P} (X - Y > 0)$
However the marginal distributions of $X sim mathcal{N} left(mu, sigma_1^2right)$ and $Y sim mathcal{N} left(mu, sigma_2^2right)$ may be different. This result holds regardless of whether $X$ and $Y$ are independent.
joint-distribution iid symmetry
$endgroup$
add a comment |
$begingroup$
I've two related propositions which seem correct intuitively, but I struggle to prove them properly.
Question 1
Prove or disprove: If $X$ and $Y$ are independent and have identical marginal distributions, then $mathbb{P} (Y > X) = mathbb{P} (X > Y) = 1/2$
Due to independence, the joint PDF of $X$ and $Y$ is the product of their marginal PDF:
$$ begin{align}
mathbb{P} (Y > X) &= int_{-infty}^infty int_x^infty p(x) , p(y) , dy , dx \
mathbb{P} (X > Y) &= int_{-infty}^infty int_y^infty p(x) , p(y) , dx , dy
= int_{-infty}^infty int_x^infty p(y) , p(x) , dy , dx
end{align} $$
The last step is based on the fact that the integral won't change if we simply rename the integration parameters $x$ and $y$ consistently. So we have shown that $mathbb{P} (Y > X) = mathbb{P} (X > Y)$
Side note: Even if $X$ and $Y$ are dependent, this result still holds so long as their joint PDF is exchangeable i.e. $p(x, y) = p(y, x)$
Let $u = y - x$ so that
$$ mathbb{P} (Y > X) = int_{-infty}^infty int_0^infty p(x) , p(u + x) , du , dx $$
I thought of applying Fubini's theorem but it doesn't help to show that it's equal to 1/2, so maybe it's not 1/2?
Alternatively, consider that
$$ mathbb{P} (Y > X) + mathbb{P} (X > Y) + mathbb{P} (Y = X) = 1 $$
If we assume that $mathbb{P} (Y = X) = 0$ then we can conclude that $mathbb{P} (Y > X) = 1/2$. But is this assumption justified?
Question 2
Prove or disprove: If $X$ and $Y$ are independent and $mathbb{P} (Y > X) = mathbb{P} (X > Y)$, then they have identical marginal distributions. If this statement is true, then is it still true if $X$ and $Y$ are dependent?
@Xi'an provided a counter-example. Suppose that
$$ begin{bmatrix} X \ Y end{bmatrix}
sim mathcal{N} left(
begin{bmatrix} mu \ mu end{bmatrix},
begin{bmatrix} sigma_1^2 & c \ c & sigma_2^2 end{bmatrix}
right)$$
Then $X-Y$ and $Y-X$ have the same distribution: $mathcal{N} left(0, sigma_1^2 + sigma_2^2 - 2c right)$ and hence $mathbb{P} (Y - X > 0) = mathbb{P} (X - Y > 0)$
However the marginal distributions of $X sim mathcal{N} left(mu, sigma_1^2right)$ and $Y sim mathcal{N} left(mu, sigma_2^2right)$ may be different. This result holds regardless of whether $X$ and $Y$ are independent.
joint-distribution iid symmetry
$endgroup$
2
$begingroup$
One interesting technical point is that if the probability of $X=c$ is $0$ for every value of $c,$ that's not enough to entail that probabilities are given by integrating a density function. The standard counterexample is the Cantor distribution. But more to the point$,ldotsqquad$
$endgroup$
– Michael Hardy
Jan 6 at 20:23
$begingroup$
$ldots,$is that I probably wouldn't solve this problem by considering such integrals anyway.
$endgroup$
– Michael Hardy
Jan 6 at 20:23
1
$begingroup$
What if X and Y are Bernoulli? Isn’t that a counterexample to P(X=Y)=0?
$endgroup$
– The Laconic
Jan 7 at 3:03
$begingroup$
When using Fubini's theorem and a density $p(cdot)$ against the Lebesgue measure, $mathbb{P} (Y = X) = 0$, necessarily.
$endgroup$
– Xi'an
Jan 7 at 9:22
add a comment |
$begingroup$
I've two related propositions which seem correct intuitively, but I struggle to prove them properly.
Question 1
Prove or disprove: If $X$ and $Y$ are independent and have identical marginal distributions, then $mathbb{P} (Y > X) = mathbb{P} (X > Y) = 1/2$
Due to independence, the joint PDF of $X$ and $Y$ is the product of their marginal PDF:
$$ begin{align}
mathbb{P} (Y > X) &= int_{-infty}^infty int_x^infty p(x) , p(y) , dy , dx \
mathbb{P} (X > Y) &= int_{-infty}^infty int_y^infty p(x) , p(y) , dx , dy
= int_{-infty}^infty int_x^infty p(y) , p(x) , dy , dx
end{align} $$
The last step is based on the fact that the integral won't change if we simply rename the integration parameters $x$ and $y$ consistently. So we have shown that $mathbb{P} (Y > X) = mathbb{P} (X > Y)$
Side note: Even if $X$ and $Y$ are dependent, this result still holds so long as their joint PDF is exchangeable i.e. $p(x, y) = p(y, x)$
Let $u = y - x$ so that
$$ mathbb{P} (Y > X) = int_{-infty}^infty int_0^infty p(x) , p(u + x) , du , dx $$
I thought of applying Fubini's theorem but it doesn't help to show that it's equal to 1/2, so maybe it's not 1/2?
Alternatively, consider that
$$ mathbb{P} (Y > X) + mathbb{P} (X > Y) + mathbb{P} (Y = X) = 1 $$
If we assume that $mathbb{P} (Y = X) = 0$ then we can conclude that $mathbb{P} (Y > X) = 1/2$. But is this assumption justified?
Question 2
Prove or disprove: If $X$ and $Y$ are independent and $mathbb{P} (Y > X) = mathbb{P} (X > Y)$, then they have identical marginal distributions. If this statement is true, then is it still true if $X$ and $Y$ are dependent?
@Xi'an provided a counter-example. Suppose that
$$ begin{bmatrix} X \ Y end{bmatrix}
sim mathcal{N} left(
begin{bmatrix} mu \ mu end{bmatrix},
begin{bmatrix} sigma_1^2 & c \ c & sigma_2^2 end{bmatrix}
right)$$
Then $X-Y$ and $Y-X$ have the same distribution: $mathcal{N} left(0, sigma_1^2 + sigma_2^2 - 2c right)$ and hence $mathbb{P} (Y - X > 0) = mathbb{P} (X - Y > 0)$
However the marginal distributions of $X sim mathcal{N} left(mu, sigma_1^2right)$ and $Y sim mathcal{N} left(mu, sigma_2^2right)$ may be different. This result holds regardless of whether $X$ and $Y$ are independent.
joint-distribution iid symmetry
$endgroup$
I've two related propositions which seem correct intuitively, but I struggle to prove them properly.
Question 1
Prove or disprove: If $X$ and $Y$ are independent and have identical marginal distributions, then $mathbb{P} (Y > X) = mathbb{P} (X > Y) = 1/2$
Due to independence, the joint PDF of $X$ and $Y$ is the product of their marginal PDF:
$$ begin{align}
mathbb{P} (Y > X) &= int_{-infty}^infty int_x^infty p(x) , p(y) , dy , dx \
mathbb{P} (X > Y) &= int_{-infty}^infty int_y^infty p(x) , p(y) , dx , dy
= int_{-infty}^infty int_x^infty p(y) , p(x) , dy , dx
end{align} $$
The last step is based on the fact that the integral won't change if we simply rename the integration parameters $x$ and $y$ consistently. So we have shown that $mathbb{P} (Y > X) = mathbb{P} (X > Y)$
Side note: Even if $X$ and $Y$ are dependent, this result still holds so long as their joint PDF is exchangeable i.e. $p(x, y) = p(y, x)$
Let $u = y - x$ so that
$$ mathbb{P} (Y > X) = int_{-infty}^infty int_0^infty p(x) , p(u + x) , du , dx $$
I thought of applying Fubini's theorem but it doesn't help to show that it's equal to 1/2, so maybe it's not 1/2?
Alternatively, consider that
$$ mathbb{P} (Y > X) + mathbb{P} (X > Y) + mathbb{P} (Y = X) = 1 $$
If we assume that $mathbb{P} (Y = X) = 0$ then we can conclude that $mathbb{P} (Y > X) = 1/2$. But is this assumption justified?
Question 2
Prove or disprove: If $X$ and $Y$ are independent and $mathbb{P} (Y > X) = mathbb{P} (X > Y)$, then they have identical marginal distributions. If this statement is true, then is it still true if $X$ and $Y$ are dependent?
@Xi'an provided a counter-example. Suppose that
$$ begin{bmatrix} X \ Y end{bmatrix}
sim mathcal{N} left(
begin{bmatrix} mu \ mu end{bmatrix},
begin{bmatrix} sigma_1^2 & c \ c & sigma_2^2 end{bmatrix}
right)$$
Then $X-Y$ and $Y-X$ have the same distribution: $mathcal{N} left(0, sigma_1^2 + sigma_2^2 - 2c right)$ and hence $mathbb{P} (Y - X > 0) = mathbb{P} (X - Y > 0)$
However the marginal distributions of $X sim mathcal{N} left(mu, sigma_1^2right)$ and $Y sim mathcal{N} left(mu, sigma_2^2right)$ may be different. This result holds regardless of whether $X$ and $Y$ are independent.
joint-distribution iid symmetry
joint-distribution iid symmetry
edited Jan 7 at 3:04
farmer
asked Jan 6 at 20:17
farmerfarmer
707
707
2
$begingroup$
One interesting technical point is that if the probability of $X=c$ is $0$ for every value of $c,$ that's not enough to entail that probabilities are given by integrating a density function. The standard counterexample is the Cantor distribution. But more to the point$,ldotsqquad$
$endgroup$
– Michael Hardy
Jan 6 at 20:23
$begingroup$
$ldots,$is that I probably wouldn't solve this problem by considering such integrals anyway.
$endgroup$
– Michael Hardy
Jan 6 at 20:23
1
$begingroup$
What if X and Y are Bernoulli? Isn’t that a counterexample to P(X=Y)=0?
$endgroup$
– The Laconic
Jan 7 at 3:03
$begingroup$
When using Fubini's theorem and a density $p(cdot)$ against the Lebesgue measure, $mathbb{P} (Y = X) = 0$, necessarily.
$endgroup$
– Xi'an
Jan 7 at 9:22
add a comment |
2
$begingroup$
One interesting technical point is that if the probability of $X=c$ is $0$ for every value of $c,$ that's not enough to entail that probabilities are given by integrating a density function. The standard counterexample is the Cantor distribution. But more to the point$,ldotsqquad$
$endgroup$
– Michael Hardy
Jan 6 at 20:23
$begingroup$
$ldots,$is that I probably wouldn't solve this problem by considering such integrals anyway.
$endgroup$
– Michael Hardy
Jan 6 at 20:23
1
$begingroup$
What if X and Y are Bernoulli? Isn’t that a counterexample to P(X=Y)=0?
$endgroup$
– The Laconic
Jan 7 at 3:03
$begingroup$
When using Fubini's theorem and a density $p(cdot)$ against the Lebesgue measure, $mathbb{P} (Y = X) = 0$, necessarily.
$endgroup$
– Xi'an
Jan 7 at 9:22
2
2
$begingroup$
One interesting technical point is that if the probability of $X=c$ is $0$ for every value of $c,$ that's not enough to entail that probabilities are given by integrating a density function. The standard counterexample is the Cantor distribution. But more to the point$,ldotsqquad$
$endgroup$
– Michael Hardy
Jan 6 at 20:23
$begingroup$
One interesting technical point is that if the probability of $X=c$ is $0$ for every value of $c,$ that's not enough to entail that probabilities are given by integrating a density function. The standard counterexample is the Cantor distribution. But more to the point$,ldotsqquad$
$endgroup$
– Michael Hardy
Jan 6 at 20:23
$begingroup$
$ldots,$is that I probably wouldn't solve this problem by considering such integrals anyway.
$endgroup$
– Michael Hardy
Jan 6 at 20:23
$begingroup$
$ldots,$is that I probably wouldn't solve this problem by considering such integrals anyway.
$endgroup$
– Michael Hardy
Jan 6 at 20:23
1
1
$begingroup$
What if X and Y are Bernoulli? Isn’t that a counterexample to P(X=Y)=0?
$endgroup$
– The Laconic
Jan 7 at 3:03
$begingroup$
What if X and Y are Bernoulli? Isn’t that a counterexample to P(X=Y)=0?
$endgroup$
– The Laconic
Jan 7 at 3:03
$begingroup$
When using Fubini's theorem and a density $p(cdot)$ against the Lebesgue measure, $mathbb{P} (Y = X) = 0$, necessarily.
$endgroup$
– Xi'an
Jan 7 at 9:22
$begingroup$
When using Fubini's theorem and a density $p(cdot)$ against the Lebesgue measure, $mathbb{P} (Y = X) = 0$, necessarily.
$endgroup$
– Xi'an
Jan 7 at 9:22
add a comment |
2 Answers
2
active
oldest
votes
$begingroup$
This answer is written under the assumption that $mathbb{P}(Y=X)=0$
which was part of the original wording of the question.
Question 1: A sufficient condition for$$mathbb{P}(X<Y)=mathbb{P}(Y<X)tag{1}$$is that $X$ and $Y$ are exchangeable, that is, that $(X,Y)$ and $(Y,X)$ have the same joint distribution. And obviously
$$mathbb{P}(X<Y)=mathbb{P}(Y<X)=1/2$$since they sum up to one. (In the alternative case that $mathbb{P}(Y=X)>0$ this is obviously no longer true.)
Question 2: Take a bivariate normal vector $(X,Y)$ with mean $(mu,mu)$. Then $X-Y$ and $Y-X$ are identically distributed, no matter what the correlation between $X$ and $Y$, and no matter what the variances of $X$ and $Y$ are, and therefore (1) holds. The conjecture is thus false.
$endgroup$
add a comment |
$begingroup$
I will show that the distribution of the pair $(X,Y)$ is the same as the distribution of the pair $(Y,X).$
That two random variables $X,Y$ are independent means that for every pair of measurable sets $A,B$ the events $[Xin A], [Yin B]$ are independent. In particular for any two numbers $x,y$ the events $[Xle x], [Yle y]$ are independent, so $F_{X,Y}(x,y) = F_X(x)cdot F_Y(y).$
And the distribution of the pair $(X,Y)$ is completely determined by the joint c.d.f.
Since it is given that $F_X=F_Y,$ we can write $F_{X,Y}(x,y) = F_X(x)cdot F_X(y).$
This is symmetric as a function of $x$ and $y,$ i.e. it remains the same if $x$ and $y$ are interchanged.
But interchanging $x$ and $y$ in $F_{X,Y}(x,y)$ is the same as interchanging $X$ and $Y,$ since
$$
F_{X,Y}(x,y) = Pr(Xle x & Yle y).
$$
Therefore (the main point):
The distribution of the pair $(X,Y)$ is the same as the distribution of the pair $(Y,X).$
$endgroup$
$begingroup$
I don't think "interchanging x and y in $F_{X,Y} (x,y)$ is the same as interchanging X and Y" because P(X ≤ x, Y ≤ y) ≠ P(X ≤ y, Y ≤ x) in general. Even if X and Y are independent, P(X ≤ x) P(Y ≤ y) ≠ P(X ≤ y) P(Y ≤ x) unless they are also identically distributed.
$endgroup$
– farmer
Jan 7 at 21:39
1
$begingroup$
@farmer : Start with $Pr(Xle x & Yle y)$ and interchange $x$ and $y,$ and you get $Pr(Xle y & Yle x).$ But if you start with the same thing and interchange $X$ and $Y,$ then you get $Pr(Yle x & Xle y).$ The claim, then, is that $Pr(Xle y & Yle x)$ is the same as $Pr(Yle x & Xle y). qquad$
$endgroup$
– Michael Hardy
Jan 8 at 5:28
add a comment |
Your Answer
StackExchange.ifUsing("editor", function () {
return StackExchange.using("mathjaxEditing", function () {
StackExchange.MarkdownEditor.creationCallbacks.add(function (editor, postfix) {
StackExchange.mathjaxEditing.prepareWmdForMathJax(editor, postfix, [["$", "$"], ["\\(","\\)"]]);
});
});
}, "mathjax-editing");
StackExchange.ready(function() {
var channelOptions = {
tags: "".split(" "),
id: "65"
};
initTagRenderer("".split(" "), "".split(" "), channelOptions);
StackExchange.using("externalEditor", function() {
// Have to fire editor after snippets, if snippets enabled
if (StackExchange.settings.snippets.snippetsEnabled) {
StackExchange.using("snippets", function() {
createEditor();
});
}
else {
createEditor();
}
});
function createEditor() {
StackExchange.prepareEditor({
heartbeatType: 'answer',
autoActivateHeartbeat: false,
convertImagesToLinks: false,
noModals: true,
showLowRepImageUploadWarning: true,
reputationToPostImages: null,
bindNavPrevention: true,
postfix: "",
imageUploader: {
brandingHtml: "Powered by u003ca class="icon-imgur-white" href="https://imgur.com/"u003eu003c/au003e",
contentPolicyHtml: "User contributions licensed under u003ca href="https://creativecommons.org/licenses/by-sa/3.0/"u003ecc by-sa 3.0 with attribution requiredu003c/au003e u003ca href="https://stackoverflow.com/legal/content-policy"u003e(content policy)u003c/au003e",
allowUrls: true
},
onDemand: true,
discardSelector: ".discard-answer"
,immediatelyShowMarkdownHelp:true
});
}
});
Sign up or log in
StackExchange.ready(function () {
StackExchange.helpers.onClickDraftSave('#login-link');
});
Sign up using Google
Sign up using Facebook
Sign up using Email and Password
Post as a guest
Required, but never shown
StackExchange.ready(
function () {
StackExchange.openid.initPostLogin('.new-post-login', 'https%3a%2f%2fstats.stackexchange.com%2fquestions%2f385885%2fidentically-distributed-vs-px-y-py-x%23new-answer', 'question_page');
}
);
Post as a guest
Required, but never shown
2 Answers
2
active
oldest
votes
2 Answers
2
active
oldest
votes
active
oldest
votes
active
oldest
votes
$begingroup$
This answer is written under the assumption that $mathbb{P}(Y=X)=0$
which was part of the original wording of the question.
Question 1: A sufficient condition for$$mathbb{P}(X<Y)=mathbb{P}(Y<X)tag{1}$$is that $X$ and $Y$ are exchangeable, that is, that $(X,Y)$ and $(Y,X)$ have the same joint distribution. And obviously
$$mathbb{P}(X<Y)=mathbb{P}(Y<X)=1/2$$since they sum up to one. (In the alternative case that $mathbb{P}(Y=X)>0$ this is obviously no longer true.)
Question 2: Take a bivariate normal vector $(X,Y)$ with mean $(mu,mu)$. Then $X-Y$ and $Y-X$ are identically distributed, no matter what the correlation between $X$ and $Y$, and no matter what the variances of $X$ and $Y$ are, and therefore (1) holds. The conjecture is thus false.
$endgroup$
add a comment |
$begingroup$
This answer is written under the assumption that $mathbb{P}(Y=X)=0$
which was part of the original wording of the question.
Question 1: A sufficient condition for$$mathbb{P}(X<Y)=mathbb{P}(Y<X)tag{1}$$is that $X$ and $Y$ are exchangeable, that is, that $(X,Y)$ and $(Y,X)$ have the same joint distribution. And obviously
$$mathbb{P}(X<Y)=mathbb{P}(Y<X)=1/2$$since they sum up to one. (In the alternative case that $mathbb{P}(Y=X)>0$ this is obviously no longer true.)
Question 2: Take a bivariate normal vector $(X,Y)$ with mean $(mu,mu)$. Then $X-Y$ and $Y-X$ are identically distributed, no matter what the correlation between $X$ and $Y$, and no matter what the variances of $X$ and $Y$ are, and therefore (1) holds. The conjecture is thus false.
$endgroup$
add a comment |
$begingroup$
This answer is written under the assumption that $mathbb{P}(Y=X)=0$
which was part of the original wording of the question.
Question 1: A sufficient condition for$$mathbb{P}(X<Y)=mathbb{P}(Y<X)tag{1}$$is that $X$ and $Y$ are exchangeable, that is, that $(X,Y)$ and $(Y,X)$ have the same joint distribution. And obviously
$$mathbb{P}(X<Y)=mathbb{P}(Y<X)=1/2$$since they sum up to one. (In the alternative case that $mathbb{P}(Y=X)>0$ this is obviously no longer true.)
Question 2: Take a bivariate normal vector $(X,Y)$ with mean $(mu,mu)$. Then $X-Y$ and $Y-X$ are identically distributed, no matter what the correlation between $X$ and $Y$, and no matter what the variances of $X$ and $Y$ are, and therefore (1) holds. The conjecture is thus false.
$endgroup$
This answer is written under the assumption that $mathbb{P}(Y=X)=0$
which was part of the original wording of the question.
Question 1: A sufficient condition for$$mathbb{P}(X<Y)=mathbb{P}(Y<X)tag{1}$$is that $X$ and $Y$ are exchangeable, that is, that $(X,Y)$ and $(Y,X)$ have the same joint distribution. And obviously
$$mathbb{P}(X<Y)=mathbb{P}(Y<X)=1/2$$since they sum up to one. (In the alternative case that $mathbb{P}(Y=X)>0$ this is obviously no longer true.)
Question 2: Take a bivariate normal vector $(X,Y)$ with mean $(mu,mu)$. Then $X-Y$ and $Y-X$ are identically distributed, no matter what the correlation between $X$ and $Y$, and no matter what the variances of $X$ and $Y$ are, and therefore (1) holds. The conjecture is thus false.
edited Jan 7 at 21:06
answered Jan 6 at 21:17


Xi'anXi'an
58.7k897363
58.7k897363
add a comment |
add a comment |
$begingroup$
I will show that the distribution of the pair $(X,Y)$ is the same as the distribution of the pair $(Y,X).$
That two random variables $X,Y$ are independent means that for every pair of measurable sets $A,B$ the events $[Xin A], [Yin B]$ are independent. In particular for any two numbers $x,y$ the events $[Xle x], [Yle y]$ are independent, so $F_{X,Y}(x,y) = F_X(x)cdot F_Y(y).$
And the distribution of the pair $(X,Y)$ is completely determined by the joint c.d.f.
Since it is given that $F_X=F_Y,$ we can write $F_{X,Y}(x,y) = F_X(x)cdot F_X(y).$
This is symmetric as a function of $x$ and $y,$ i.e. it remains the same if $x$ and $y$ are interchanged.
But interchanging $x$ and $y$ in $F_{X,Y}(x,y)$ is the same as interchanging $X$ and $Y,$ since
$$
F_{X,Y}(x,y) = Pr(Xle x & Yle y).
$$
Therefore (the main point):
The distribution of the pair $(X,Y)$ is the same as the distribution of the pair $(Y,X).$
$endgroup$
$begingroup$
I don't think "interchanging x and y in $F_{X,Y} (x,y)$ is the same as interchanging X and Y" because P(X ≤ x, Y ≤ y) ≠ P(X ≤ y, Y ≤ x) in general. Even if X and Y are independent, P(X ≤ x) P(Y ≤ y) ≠ P(X ≤ y) P(Y ≤ x) unless they are also identically distributed.
$endgroup$
– farmer
Jan 7 at 21:39
1
$begingroup$
@farmer : Start with $Pr(Xle x & Yle y)$ and interchange $x$ and $y,$ and you get $Pr(Xle y & Yle x).$ But if you start with the same thing and interchange $X$ and $Y,$ then you get $Pr(Yle x & Xle y).$ The claim, then, is that $Pr(Xle y & Yle x)$ is the same as $Pr(Yle x & Xle y). qquad$
$endgroup$
– Michael Hardy
Jan 8 at 5:28
add a comment |
$begingroup$
I will show that the distribution of the pair $(X,Y)$ is the same as the distribution of the pair $(Y,X).$
That two random variables $X,Y$ are independent means that for every pair of measurable sets $A,B$ the events $[Xin A], [Yin B]$ are independent. In particular for any two numbers $x,y$ the events $[Xle x], [Yle y]$ are independent, so $F_{X,Y}(x,y) = F_X(x)cdot F_Y(y).$
And the distribution of the pair $(X,Y)$ is completely determined by the joint c.d.f.
Since it is given that $F_X=F_Y,$ we can write $F_{X,Y}(x,y) = F_X(x)cdot F_X(y).$
This is symmetric as a function of $x$ and $y,$ i.e. it remains the same if $x$ and $y$ are interchanged.
But interchanging $x$ and $y$ in $F_{X,Y}(x,y)$ is the same as interchanging $X$ and $Y,$ since
$$
F_{X,Y}(x,y) = Pr(Xle x & Yle y).
$$
Therefore (the main point):
The distribution of the pair $(X,Y)$ is the same as the distribution of the pair $(Y,X).$
$endgroup$
$begingroup$
I don't think "interchanging x and y in $F_{X,Y} (x,y)$ is the same as interchanging X and Y" because P(X ≤ x, Y ≤ y) ≠ P(X ≤ y, Y ≤ x) in general. Even if X and Y are independent, P(X ≤ x) P(Y ≤ y) ≠ P(X ≤ y) P(Y ≤ x) unless they are also identically distributed.
$endgroup$
– farmer
Jan 7 at 21:39
1
$begingroup$
@farmer : Start with $Pr(Xle x & Yle y)$ and interchange $x$ and $y,$ and you get $Pr(Xle y & Yle x).$ But if you start with the same thing and interchange $X$ and $Y,$ then you get $Pr(Yle x & Xle y).$ The claim, then, is that $Pr(Xle y & Yle x)$ is the same as $Pr(Yle x & Xle y). qquad$
$endgroup$
– Michael Hardy
Jan 8 at 5:28
add a comment |
$begingroup$
I will show that the distribution of the pair $(X,Y)$ is the same as the distribution of the pair $(Y,X).$
That two random variables $X,Y$ are independent means that for every pair of measurable sets $A,B$ the events $[Xin A], [Yin B]$ are independent. In particular for any two numbers $x,y$ the events $[Xle x], [Yle y]$ are independent, so $F_{X,Y}(x,y) = F_X(x)cdot F_Y(y).$
And the distribution of the pair $(X,Y)$ is completely determined by the joint c.d.f.
Since it is given that $F_X=F_Y,$ we can write $F_{X,Y}(x,y) = F_X(x)cdot F_X(y).$
This is symmetric as a function of $x$ and $y,$ i.e. it remains the same if $x$ and $y$ are interchanged.
But interchanging $x$ and $y$ in $F_{X,Y}(x,y)$ is the same as interchanging $X$ and $Y,$ since
$$
F_{X,Y}(x,y) = Pr(Xle x & Yle y).
$$
Therefore (the main point):
The distribution of the pair $(X,Y)$ is the same as the distribution of the pair $(Y,X).$
$endgroup$
I will show that the distribution of the pair $(X,Y)$ is the same as the distribution of the pair $(Y,X).$
That two random variables $X,Y$ are independent means that for every pair of measurable sets $A,B$ the events $[Xin A], [Yin B]$ are independent. In particular for any two numbers $x,y$ the events $[Xle x], [Yle y]$ are independent, so $F_{X,Y}(x,y) = F_X(x)cdot F_Y(y).$
And the distribution of the pair $(X,Y)$ is completely determined by the joint c.d.f.
Since it is given that $F_X=F_Y,$ we can write $F_{X,Y}(x,y) = F_X(x)cdot F_X(y).$
This is symmetric as a function of $x$ and $y,$ i.e. it remains the same if $x$ and $y$ are interchanged.
But interchanging $x$ and $y$ in $F_{X,Y}(x,y)$ is the same as interchanging $X$ and $Y,$ since
$$
F_{X,Y}(x,y) = Pr(Xle x & Yle y).
$$
Therefore (the main point):
The distribution of the pair $(X,Y)$ is the same as the distribution of the pair $(Y,X).$
edited Jan 6 at 21:52
answered Jan 6 at 21:46
Michael HardyMichael Hardy
3,9151430
3,9151430
$begingroup$
I don't think "interchanging x and y in $F_{X,Y} (x,y)$ is the same as interchanging X and Y" because P(X ≤ x, Y ≤ y) ≠ P(X ≤ y, Y ≤ x) in general. Even if X and Y are independent, P(X ≤ x) P(Y ≤ y) ≠ P(X ≤ y) P(Y ≤ x) unless they are also identically distributed.
$endgroup$
– farmer
Jan 7 at 21:39
1
$begingroup$
@farmer : Start with $Pr(Xle x & Yle y)$ and interchange $x$ and $y,$ and you get $Pr(Xle y & Yle x).$ But if you start with the same thing and interchange $X$ and $Y,$ then you get $Pr(Yle x & Xle y).$ The claim, then, is that $Pr(Xle y & Yle x)$ is the same as $Pr(Yle x & Xle y). qquad$
$endgroup$
– Michael Hardy
Jan 8 at 5:28
add a comment |
$begingroup$
I don't think "interchanging x and y in $F_{X,Y} (x,y)$ is the same as interchanging X and Y" because P(X ≤ x, Y ≤ y) ≠ P(X ≤ y, Y ≤ x) in general. Even if X and Y are independent, P(X ≤ x) P(Y ≤ y) ≠ P(X ≤ y) P(Y ≤ x) unless they are also identically distributed.
$endgroup$
– farmer
Jan 7 at 21:39
1
$begingroup$
@farmer : Start with $Pr(Xle x & Yle y)$ and interchange $x$ and $y,$ and you get $Pr(Xle y & Yle x).$ But if you start with the same thing and interchange $X$ and $Y,$ then you get $Pr(Yle x & Xle y).$ The claim, then, is that $Pr(Xle y & Yle x)$ is the same as $Pr(Yle x & Xle y). qquad$
$endgroup$
– Michael Hardy
Jan 8 at 5:28
$begingroup$
I don't think "interchanging x and y in $F_{X,Y} (x,y)$ is the same as interchanging X and Y" because P(X ≤ x, Y ≤ y) ≠ P(X ≤ y, Y ≤ x) in general. Even if X and Y are independent, P(X ≤ x) P(Y ≤ y) ≠ P(X ≤ y) P(Y ≤ x) unless they are also identically distributed.
$endgroup$
– farmer
Jan 7 at 21:39
$begingroup$
I don't think "interchanging x and y in $F_{X,Y} (x,y)$ is the same as interchanging X and Y" because P(X ≤ x, Y ≤ y) ≠ P(X ≤ y, Y ≤ x) in general. Even if X and Y are independent, P(X ≤ x) P(Y ≤ y) ≠ P(X ≤ y) P(Y ≤ x) unless they are also identically distributed.
$endgroup$
– farmer
Jan 7 at 21:39
1
1
$begingroup$
@farmer : Start with $Pr(Xle x & Yle y)$ and interchange $x$ and $y,$ and you get $Pr(Xle y & Yle x).$ But if you start with the same thing and interchange $X$ and $Y,$ then you get $Pr(Yle x & Xle y).$ The claim, then, is that $Pr(Xle y & Yle x)$ is the same as $Pr(Yle x & Xle y). qquad$
$endgroup$
– Michael Hardy
Jan 8 at 5:28
$begingroup$
@farmer : Start with $Pr(Xle x & Yle y)$ and interchange $x$ and $y,$ and you get $Pr(Xle y & Yle x).$ But if you start with the same thing and interchange $X$ and $Y,$ then you get $Pr(Yle x & Xle y).$ The claim, then, is that $Pr(Xle y & Yle x)$ is the same as $Pr(Yle x & Xle y). qquad$
$endgroup$
– Michael Hardy
Jan 8 at 5:28
add a comment |
Thanks for contributing an answer to Cross Validated!
- Please be sure to answer the question. Provide details and share your research!
But avoid …
- Asking for help, clarification, or responding to other answers.
- Making statements based on opinion; back them up with references or personal experience.
Use MathJax to format equations. MathJax reference.
To learn more, see our tips on writing great answers.
Sign up or log in
StackExchange.ready(function () {
StackExchange.helpers.onClickDraftSave('#login-link');
});
Sign up using Google
Sign up using Facebook
Sign up using Email and Password
Post as a guest
Required, but never shown
StackExchange.ready(
function () {
StackExchange.openid.initPostLogin('.new-post-login', 'https%3a%2f%2fstats.stackexchange.com%2fquestions%2f385885%2fidentically-distributed-vs-px-y-py-x%23new-answer', 'question_page');
}
);
Post as a guest
Required, but never shown
Sign up or log in
StackExchange.ready(function () {
StackExchange.helpers.onClickDraftSave('#login-link');
});
Sign up using Google
Sign up using Facebook
Sign up using Email and Password
Post as a guest
Required, but never shown
Sign up or log in
StackExchange.ready(function () {
StackExchange.helpers.onClickDraftSave('#login-link');
});
Sign up using Google
Sign up using Facebook
Sign up using Email and Password
Post as a guest
Required, but never shown
Sign up or log in
StackExchange.ready(function () {
StackExchange.helpers.onClickDraftSave('#login-link');
});
Sign up using Google
Sign up using Facebook
Sign up using Email and Password
Sign up using Google
Sign up using Facebook
Sign up using Email and Password
Post as a guest
Required, but never shown
Required, but never shown
Required, but never shown
Required, but never shown
Required, but never shown
Required, but never shown
Required, but never shown
Required, but never shown
Required, but never shown
2dpNzquQI,lCrzfAXamL
2
$begingroup$
One interesting technical point is that if the probability of $X=c$ is $0$ for every value of $c,$ that's not enough to entail that probabilities are given by integrating a density function. The standard counterexample is the Cantor distribution. But more to the point$,ldotsqquad$
$endgroup$
– Michael Hardy
Jan 6 at 20:23
$begingroup$
$ldots,$is that I probably wouldn't solve this problem by considering such integrals anyway.
$endgroup$
– Michael Hardy
Jan 6 at 20:23
1
$begingroup$
What if X and Y are Bernoulli? Isn’t that a counterexample to P(X=Y)=0?
$endgroup$
– The Laconic
Jan 7 at 3:03
$begingroup$
When using Fubini's theorem and a density $p(cdot)$ against the Lebesgue measure, $mathbb{P} (Y = X) = 0$, necessarily.
$endgroup$
– Xi'an
Jan 7 at 9:22