Computing marginal distribution
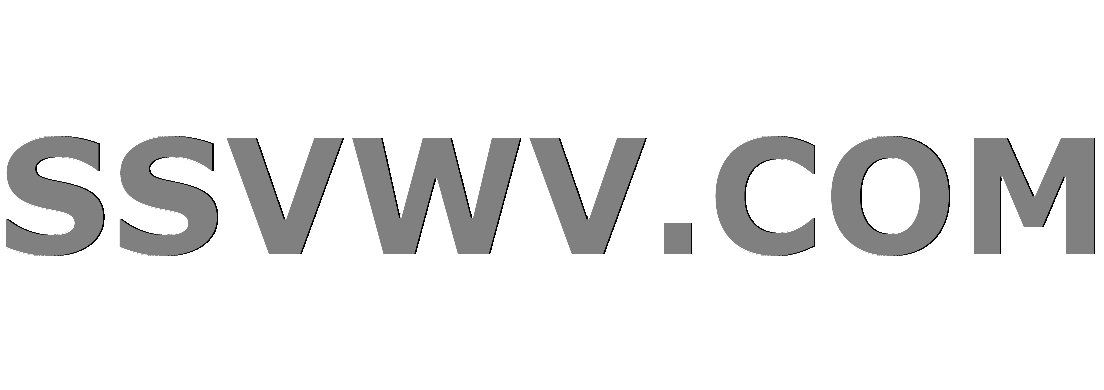
Multi tool use
$begingroup$
Assume $x$ is distributed with $F(x)=x^2$ for $0leq x leq 1$ and $cmid x sim U[0, lambda cdot x + 1-lambda]$ for some $0 < lambda < 1$. I am trying to find the marginal distribution of c.
The joint density function should be given by $frac{2x}{lambda cdot x + 1-lambda}$ whenever $x in [0,1]$ and $c in [0, lambda cdot x + 1- lambda]$, right?
I tried integrating out $x$ next, but I am unsure about the boundaries. Simply letting $x geq frac{c+lambda -1}{lambda}$ seems not to be correct, as this lower bound could be negative?
I think should be getting a marginal density for $c$ that integrates to 1 on the support $[0,1]$, regardless of what value $lambda$ takes, but it never worked no matter what I tried...
real-analysis probability integration probability-theory density-function
$endgroup$
add a comment |
$begingroup$
Assume $x$ is distributed with $F(x)=x^2$ for $0leq x leq 1$ and $cmid x sim U[0, lambda cdot x + 1-lambda]$ for some $0 < lambda < 1$. I am trying to find the marginal distribution of c.
The joint density function should be given by $frac{2x}{lambda cdot x + 1-lambda}$ whenever $x in [0,1]$ and $c in [0, lambda cdot x + 1- lambda]$, right?
I tried integrating out $x$ next, but I am unsure about the boundaries. Simply letting $x geq frac{c+lambda -1}{lambda}$ seems not to be correct, as this lower bound could be negative?
I think should be getting a marginal density for $c$ that integrates to 1 on the support $[0,1]$, regardless of what value $lambda$ takes, but it never worked no matter what I tried...
real-analysis probability integration probability-theory density-function
$endgroup$
$begingroup$
Sometimes it is helpful to take a random sample from the joint distribution (randomly selecting an x followed by a value of c conditional on x) and plot the (x,c) pairs along with a histogram of the resulting c values.
$endgroup$
– JimB
Jan 11 at 7:03
$begingroup$
Another hint: Look at the joint distribution and first find $Pr(C leq c)$ as a piecewise function which depends on $c$ being above or below $1-lambda$. Then differentiate the two pieces to get the marginal pdf for $C$.
$endgroup$
– JimB
Jan 11 at 17:07
add a comment |
$begingroup$
Assume $x$ is distributed with $F(x)=x^2$ for $0leq x leq 1$ and $cmid x sim U[0, lambda cdot x + 1-lambda]$ for some $0 < lambda < 1$. I am trying to find the marginal distribution of c.
The joint density function should be given by $frac{2x}{lambda cdot x + 1-lambda}$ whenever $x in [0,1]$ and $c in [0, lambda cdot x + 1- lambda]$, right?
I tried integrating out $x$ next, but I am unsure about the boundaries. Simply letting $x geq frac{c+lambda -1}{lambda}$ seems not to be correct, as this lower bound could be negative?
I think should be getting a marginal density for $c$ that integrates to 1 on the support $[0,1]$, regardless of what value $lambda$ takes, but it never worked no matter what I tried...
real-analysis probability integration probability-theory density-function
$endgroup$
Assume $x$ is distributed with $F(x)=x^2$ for $0leq x leq 1$ and $cmid x sim U[0, lambda cdot x + 1-lambda]$ for some $0 < lambda < 1$. I am trying to find the marginal distribution of c.
The joint density function should be given by $frac{2x}{lambda cdot x + 1-lambda}$ whenever $x in [0,1]$ and $c in [0, lambda cdot x + 1- lambda]$, right?
I tried integrating out $x$ next, but I am unsure about the boundaries. Simply letting $x geq frac{c+lambda -1}{lambda}$ seems not to be correct, as this lower bound could be negative?
I think should be getting a marginal density for $c$ that integrates to 1 on the support $[0,1]$, regardless of what value $lambda$ takes, but it never worked no matter what I tried...
real-analysis probability integration probability-theory density-function
real-analysis probability integration probability-theory density-function
asked Jan 11 at 3:39
user509037user509037
836
836
$begingroup$
Sometimes it is helpful to take a random sample from the joint distribution (randomly selecting an x followed by a value of c conditional on x) and plot the (x,c) pairs along with a histogram of the resulting c values.
$endgroup$
– JimB
Jan 11 at 7:03
$begingroup$
Another hint: Look at the joint distribution and first find $Pr(C leq c)$ as a piecewise function which depends on $c$ being above or below $1-lambda$. Then differentiate the two pieces to get the marginal pdf for $C$.
$endgroup$
– JimB
Jan 11 at 17:07
add a comment |
$begingroup$
Sometimes it is helpful to take a random sample from the joint distribution (randomly selecting an x followed by a value of c conditional on x) and plot the (x,c) pairs along with a histogram of the resulting c values.
$endgroup$
– JimB
Jan 11 at 7:03
$begingroup$
Another hint: Look at the joint distribution and first find $Pr(C leq c)$ as a piecewise function which depends on $c$ being above or below $1-lambda$. Then differentiate the two pieces to get the marginal pdf for $C$.
$endgroup$
– JimB
Jan 11 at 17:07
$begingroup$
Sometimes it is helpful to take a random sample from the joint distribution (randomly selecting an x followed by a value of c conditional on x) and plot the (x,c) pairs along with a histogram of the resulting c values.
$endgroup$
– JimB
Jan 11 at 7:03
$begingroup$
Sometimes it is helpful to take a random sample from the joint distribution (randomly selecting an x followed by a value of c conditional on x) and plot the (x,c) pairs along with a histogram of the resulting c values.
$endgroup$
– JimB
Jan 11 at 7:03
$begingroup$
Another hint: Look at the joint distribution and first find $Pr(C leq c)$ as a piecewise function which depends on $c$ being above or below $1-lambda$. Then differentiate the two pieces to get the marginal pdf for $C$.
$endgroup$
– JimB
Jan 11 at 17:07
$begingroup$
Another hint: Look at the joint distribution and first find $Pr(C leq c)$ as a piecewise function which depends on $c$ being above or below $1-lambda$. Then differentiate the two pieces to get the marginal pdf for $C$.
$endgroup$
– JimB
Jan 11 at 17:07
add a comment |
1 Answer
1
active
oldest
votes
$begingroup$
First look at the sample space associated with a non-zero joint density:
The $1-lambda$ location on the figure is for $lambda=0.3$ but it is meant to be a general y-intercept of upper boundary for $c$.
We see that to construct the value of $Pr[Cleq c_0]$ we need to consider two cases: (1) $c_0 <= 1-lambda$ and $c_0 > 1-lambda$.
$$Pr[Cleq c_0 | 0 leq c_0leq 1-lambda leq 1]=int _0^1int _0^{c_0}frac{2 x}{1-lambda (1-x)} dc dx = frac{2 c_0 (lambda -(lambda -1) log (1-lambda ))}{lambda ^2}$$
$$Pr[Cleq c_0 | 0 lt 1-lambda < c_0 leq 1]=\ int _0^{frac{c_0+lambda -1}{lambda }}int _0^{1-lambda (1-x)}frac{2 x}{1-lambda (1-x)}dcdx+int _{frac{c_0+lambda -1}{lambda }}^1int _0^{c_0}frac{2 x}{1-lambda (1-x)}dcdx =\
frac{(c_0+lambda -1)^2-2 c_0 ((lambda -1) log (c_0)+c_0-1)}{lambda ^2}$$
So
$$Pr[Cleq c_0 | 0 lt 1-lambda < c_0 leq 1]=begin{array}{cc}
{ &
begin{array}{cc}
frac{2 c_0 (lambda -(lambda -1) log (1-lambda ))}{lambda ^2} & 0leq c_0leq 1-lambda <1 \
frac{(c_0+lambda -1)^2-2 c_0 ((lambda -1) log (c_0)+c_0-1)}{lambda ^2} & 0<1-lambda <c_0<1 \
end{array}
\
end{array}$$
Now take the derivative with respect to $c_0$ to obtain the marginal probability density function for $C$:
$$f(c)=begin{array}{cc}
{ &
begin{array}{cc}
frac{2 (lambda -(lambda -1) log (1-lambda ))}{lambda ^2} & 0leq c_0 leq 1-lambda \
-frac{2 ((lambda -1) log (c_0)+c_0-1)}{lambda ^2} & 0<1-lambda< c_0<1 \
end{array}
\
end{array}$$
Here is what the marginal pdf for $C$ looks like for various values of $lambda$:
$endgroup$
add a comment |
Your Answer
StackExchange.ifUsing("editor", function () {
return StackExchange.using("mathjaxEditing", function () {
StackExchange.MarkdownEditor.creationCallbacks.add(function (editor, postfix) {
StackExchange.mathjaxEditing.prepareWmdForMathJax(editor, postfix, [["$", "$"], ["\\(","\\)"]]);
});
});
}, "mathjax-editing");
StackExchange.ready(function() {
var channelOptions = {
tags: "".split(" "),
id: "69"
};
initTagRenderer("".split(" "), "".split(" "), channelOptions);
StackExchange.using("externalEditor", function() {
// Have to fire editor after snippets, if snippets enabled
if (StackExchange.settings.snippets.snippetsEnabled) {
StackExchange.using("snippets", function() {
createEditor();
});
}
else {
createEditor();
}
});
function createEditor() {
StackExchange.prepareEditor({
heartbeatType: 'answer',
autoActivateHeartbeat: false,
convertImagesToLinks: true,
noModals: true,
showLowRepImageUploadWarning: true,
reputationToPostImages: 10,
bindNavPrevention: true,
postfix: "",
imageUploader: {
brandingHtml: "Powered by u003ca class="icon-imgur-white" href="https://imgur.com/"u003eu003c/au003e",
contentPolicyHtml: "User contributions licensed under u003ca href="https://creativecommons.org/licenses/by-sa/3.0/"u003ecc by-sa 3.0 with attribution requiredu003c/au003e u003ca href="https://stackoverflow.com/legal/content-policy"u003e(content policy)u003c/au003e",
allowUrls: true
},
noCode: true, onDemand: true,
discardSelector: ".discard-answer"
,immediatelyShowMarkdownHelp:true
});
}
});
Sign up or log in
StackExchange.ready(function () {
StackExchange.helpers.onClickDraftSave('#login-link');
});
Sign up using Google
Sign up using Facebook
Sign up using Email and Password
Post as a guest
Required, but never shown
StackExchange.ready(
function () {
StackExchange.openid.initPostLogin('.new-post-login', 'https%3a%2f%2fmath.stackexchange.com%2fquestions%2f3069461%2fcomputing-marginal-distribution%23new-answer', 'question_page');
}
);
Post as a guest
Required, but never shown
1 Answer
1
active
oldest
votes
1 Answer
1
active
oldest
votes
active
oldest
votes
active
oldest
votes
$begingroup$
First look at the sample space associated with a non-zero joint density:
The $1-lambda$ location on the figure is for $lambda=0.3$ but it is meant to be a general y-intercept of upper boundary for $c$.
We see that to construct the value of $Pr[Cleq c_0]$ we need to consider two cases: (1) $c_0 <= 1-lambda$ and $c_0 > 1-lambda$.
$$Pr[Cleq c_0 | 0 leq c_0leq 1-lambda leq 1]=int _0^1int _0^{c_0}frac{2 x}{1-lambda (1-x)} dc dx = frac{2 c_0 (lambda -(lambda -1) log (1-lambda ))}{lambda ^2}$$
$$Pr[Cleq c_0 | 0 lt 1-lambda < c_0 leq 1]=\ int _0^{frac{c_0+lambda -1}{lambda }}int _0^{1-lambda (1-x)}frac{2 x}{1-lambda (1-x)}dcdx+int _{frac{c_0+lambda -1}{lambda }}^1int _0^{c_0}frac{2 x}{1-lambda (1-x)}dcdx =\
frac{(c_0+lambda -1)^2-2 c_0 ((lambda -1) log (c_0)+c_0-1)}{lambda ^2}$$
So
$$Pr[Cleq c_0 | 0 lt 1-lambda < c_0 leq 1]=begin{array}{cc}
{ &
begin{array}{cc}
frac{2 c_0 (lambda -(lambda -1) log (1-lambda ))}{lambda ^2} & 0leq c_0leq 1-lambda <1 \
frac{(c_0+lambda -1)^2-2 c_0 ((lambda -1) log (c_0)+c_0-1)}{lambda ^2} & 0<1-lambda <c_0<1 \
end{array}
\
end{array}$$
Now take the derivative with respect to $c_0$ to obtain the marginal probability density function for $C$:
$$f(c)=begin{array}{cc}
{ &
begin{array}{cc}
frac{2 (lambda -(lambda -1) log (1-lambda ))}{lambda ^2} & 0leq c_0 leq 1-lambda \
-frac{2 ((lambda -1) log (c_0)+c_0-1)}{lambda ^2} & 0<1-lambda< c_0<1 \
end{array}
\
end{array}$$
Here is what the marginal pdf for $C$ looks like for various values of $lambda$:
$endgroup$
add a comment |
$begingroup$
First look at the sample space associated with a non-zero joint density:
The $1-lambda$ location on the figure is for $lambda=0.3$ but it is meant to be a general y-intercept of upper boundary for $c$.
We see that to construct the value of $Pr[Cleq c_0]$ we need to consider two cases: (1) $c_0 <= 1-lambda$ and $c_0 > 1-lambda$.
$$Pr[Cleq c_0 | 0 leq c_0leq 1-lambda leq 1]=int _0^1int _0^{c_0}frac{2 x}{1-lambda (1-x)} dc dx = frac{2 c_0 (lambda -(lambda -1) log (1-lambda ))}{lambda ^2}$$
$$Pr[Cleq c_0 | 0 lt 1-lambda < c_0 leq 1]=\ int _0^{frac{c_0+lambda -1}{lambda }}int _0^{1-lambda (1-x)}frac{2 x}{1-lambda (1-x)}dcdx+int _{frac{c_0+lambda -1}{lambda }}^1int _0^{c_0}frac{2 x}{1-lambda (1-x)}dcdx =\
frac{(c_0+lambda -1)^2-2 c_0 ((lambda -1) log (c_0)+c_0-1)}{lambda ^2}$$
So
$$Pr[Cleq c_0 | 0 lt 1-lambda < c_0 leq 1]=begin{array}{cc}
{ &
begin{array}{cc}
frac{2 c_0 (lambda -(lambda -1) log (1-lambda ))}{lambda ^2} & 0leq c_0leq 1-lambda <1 \
frac{(c_0+lambda -1)^2-2 c_0 ((lambda -1) log (c_0)+c_0-1)}{lambda ^2} & 0<1-lambda <c_0<1 \
end{array}
\
end{array}$$
Now take the derivative with respect to $c_0$ to obtain the marginal probability density function for $C$:
$$f(c)=begin{array}{cc}
{ &
begin{array}{cc}
frac{2 (lambda -(lambda -1) log (1-lambda ))}{lambda ^2} & 0leq c_0 leq 1-lambda \
-frac{2 ((lambda -1) log (c_0)+c_0-1)}{lambda ^2} & 0<1-lambda< c_0<1 \
end{array}
\
end{array}$$
Here is what the marginal pdf for $C$ looks like for various values of $lambda$:
$endgroup$
add a comment |
$begingroup$
First look at the sample space associated with a non-zero joint density:
The $1-lambda$ location on the figure is for $lambda=0.3$ but it is meant to be a general y-intercept of upper boundary for $c$.
We see that to construct the value of $Pr[Cleq c_0]$ we need to consider two cases: (1) $c_0 <= 1-lambda$ and $c_0 > 1-lambda$.
$$Pr[Cleq c_0 | 0 leq c_0leq 1-lambda leq 1]=int _0^1int _0^{c_0}frac{2 x}{1-lambda (1-x)} dc dx = frac{2 c_0 (lambda -(lambda -1) log (1-lambda ))}{lambda ^2}$$
$$Pr[Cleq c_0 | 0 lt 1-lambda < c_0 leq 1]=\ int _0^{frac{c_0+lambda -1}{lambda }}int _0^{1-lambda (1-x)}frac{2 x}{1-lambda (1-x)}dcdx+int _{frac{c_0+lambda -1}{lambda }}^1int _0^{c_0}frac{2 x}{1-lambda (1-x)}dcdx =\
frac{(c_0+lambda -1)^2-2 c_0 ((lambda -1) log (c_0)+c_0-1)}{lambda ^2}$$
So
$$Pr[Cleq c_0 | 0 lt 1-lambda < c_0 leq 1]=begin{array}{cc}
{ &
begin{array}{cc}
frac{2 c_0 (lambda -(lambda -1) log (1-lambda ))}{lambda ^2} & 0leq c_0leq 1-lambda <1 \
frac{(c_0+lambda -1)^2-2 c_0 ((lambda -1) log (c_0)+c_0-1)}{lambda ^2} & 0<1-lambda <c_0<1 \
end{array}
\
end{array}$$
Now take the derivative with respect to $c_0$ to obtain the marginal probability density function for $C$:
$$f(c)=begin{array}{cc}
{ &
begin{array}{cc}
frac{2 (lambda -(lambda -1) log (1-lambda ))}{lambda ^2} & 0leq c_0 leq 1-lambda \
-frac{2 ((lambda -1) log (c_0)+c_0-1)}{lambda ^2} & 0<1-lambda< c_0<1 \
end{array}
\
end{array}$$
Here is what the marginal pdf for $C$ looks like for various values of $lambda$:
$endgroup$
First look at the sample space associated with a non-zero joint density:
The $1-lambda$ location on the figure is for $lambda=0.3$ but it is meant to be a general y-intercept of upper boundary for $c$.
We see that to construct the value of $Pr[Cleq c_0]$ we need to consider two cases: (1) $c_0 <= 1-lambda$ and $c_0 > 1-lambda$.
$$Pr[Cleq c_0 | 0 leq c_0leq 1-lambda leq 1]=int _0^1int _0^{c_0}frac{2 x}{1-lambda (1-x)} dc dx = frac{2 c_0 (lambda -(lambda -1) log (1-lambda ))}{lambda ^2}$$
$$Pr[Cleq c_0 | 0 lt 1-lambda < c_0 leq 1]=\ int _0^{frac{c_0+lambda -1}{lambda }}int _0^{1-lambda (1-x)}frac{2 x}{1-lambda (1-x)}dcdx+int _{frac{c_0+lambda -1}{lambda }}^1int _0^{c_0}frac{2 x}{1-lambda (1-x)}dcdx =\
frac{(c_0+lambda -1)^2-2 c_0 ((lambda -1) log (c_0)+c_0-1)}{lambda ^2}$$
So
$$Pr[Cleq c_0 | 0 lt 1-lambda < c_0 leq 1]=begin{array}{cc}
{ &
begin{array}{cc}
frac{2 c_0 (lambda -(lambda -1) log (1-lambda ))}{lambda ^2} & 0leq c_0leq 1-lambda <1 \
frac{(c_0+lambda -1)^2-2 c_0 ((lambda -1) log (c_0)+c_0-1)}{lambda ^2} & 0<1-lambda <c_0<1 \
end{array}
\
end{array}$$
Now take the derivative with respect to $c_0$ to obtain the marginal probability density function for $C$:
$$f(c)=begin{array}{cc}
{ &
begin{array}{cc}
frac{2 (lambda -(lambda -1) log (1-lambda ))}{lambda ^2} & 0leq c_0 leq 1-lambda \
-frac{2 ((lambda -1) log (c_0)+c_0-1)}{lambda ^2} & 0<1-lambda< c_0<1 \
end{array}
\
end{array}$$
Here is what the marginal pdf for $C$ looks like for various values of $lambda$:
edited Jan 12 at 5:53
answered Jan 12 at 5:19
JimBJimB
61547
61547
add a comment |
add a comment |
Thanks for contributing an answer to Mathematics Stack Exchange!
- Please be sure to answer the question. Provide details and share your research!
But avoid …
- Asking for help, clarification, or responding to other answers.
- Making statements based on opinion; back them up with references or personal experience.
Use MathJax to format equations. MathJax reference.
To learn more, see our tips on writing great answers.
Sign up or log in
StackExchange.ready(function () {
StackExchange.helpers.onClickDraftSave('#login-link');
});
Sign up using Google
Sign up using Facebook
Sign up using Email and Password
Post as a guest
Required, but never shown
StackExchange.ready(
function () {
StackExchange.openid.initPostLogin('.new-post-login', 'https%3a%2f%2fmath.stackexchange.com%2fquestions%2f3069461%2fcomputing-marginal-distribution%23new-answer', 'question_page');
}
);
Post as a guest
Required, but never shown
Sign up or log in
StackExchange.ready(function () {
StackExchange.helpers.onClickDraftSave('#login-link');
});
Sign up using Google
Sign up using Facebook
Sign up using Email and Password
Post as a guest
Required, but never shown
Sign up or log in
StackExchange.ready(function () {
StackExchange.helpers.onClickDraftSave('#login-link');
});
Sign up using Google
Sign up using Facebook
Sign up using Email and Password
Post as a guest
Required, but never shown
Sign up or log in
StackExchange.ready(function () {
StackExchange.helpers.onClickDraftSave('#login-link');
});
Sign up using Google
Sign up using Facebook
Sign up using Email and Password
Sign up using Google
Sign up using Facebook
Sign up using Email and Password
Post as a guest
Required, but never shown
Required, but never shown
Required, but never shown
Required, but never shown
Required, but never shown
Required, but never shown
Required, but never shown
Required, but never shown
Required, but never shown
vJLp2BRTk7NCyxM,yuixsGl 1lT4Vbe93XxLxMw8sTwDX,3F8sS7w
$begingroup$
Sometimes it is helpful to take a random sample from the joint distribution (randomly selecting an x followed by a value of c conditional on x) and plot the (x,c) pairs along with a histogram of the resulting c values.
$endgroup$
– JimB
Jan 11 at 7:03
$begingroup$
Another hint: Look at the joint distribution and first find $Pr(C leq c)$ as a piecewise function which depends on $c$ being above or below $1-lambda$. Then differentiate the two pieces to get the marginal pdf for $C$.
$endgroup$
– JimB
Jan 11 at 17:07