Discretizing a Stochastic Volatility SDE
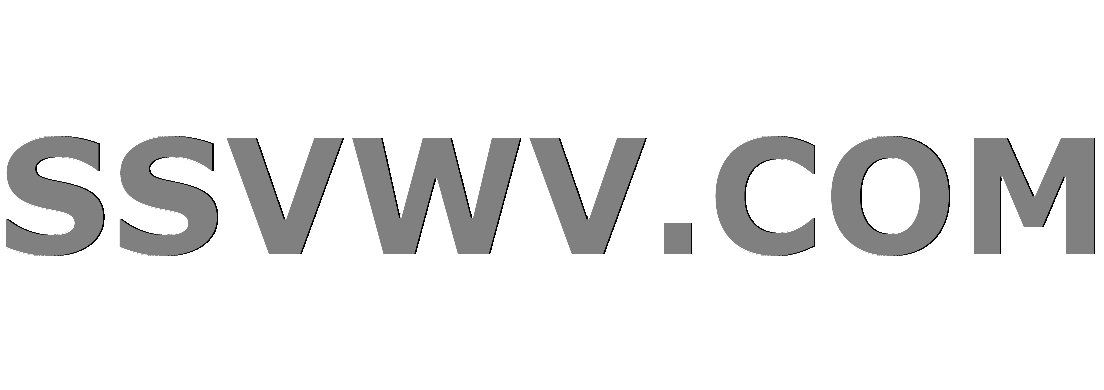
Multi tool use
$begingroup$
How does the discrete time stochastic volatility model arise from the continuous time one?
I have the following continuous time stochastic volatility model. $S_t$ is the price, and $v_t$ is a variance process.
$$
dS_t = mu S_tdt + sqrt{v_t}S_t dB_{1t} \
dv_t = (theta - alpha log v_t)v_tdt + sigma v_t dB_{2t} .
$$
I'm more familiar with the discrete time version:
$$
y_t = exp(h_t/2)epsilon_t \
h_{t+1} = mu + phi(h_t - mu) + sigma_t eta_t \
h_1 sim Nleft(mu, frac{sigma^2}{1-phi^2}right).
$$
${y_t}$ are the log returns, and ${h_t}$ are the "log-volatilites." Keep in mind there might be some confusion about parameters; for example the $mu$s in each of these models are different.
How do I verify that the first discretizes into the second?
Here's my work so far. First I define $Y_t = log S_t$ and $h_t = log v_t$. Then I use Ito's lemma to get
begin{align*}
dY_t &= left(mu - frac{exp h_t}{2}right)dt + exp[h_t/2] dB_{1t}\
dh_t &= left(theta - alphalog v_t - sigma^2/2right)dt + sigma dB_{2,t}\
&= alphaleft(tilde{mu} - h_t right)dt + sigma dB_{2t}.
end{align*}
I got the state/log-vol process piece. I use the Euler method to discretize, setting $Delta t = 1$, to get
begin{align*}
h_{t+1} &= alpha tilde{mu} + h_t(1-alpha) + sigma eta_t \
&= tilde{mu}(1 - phi) + phi h_t + sigma eta_t \
&= tilde{mu} + phi(h_t - tilde{mu}) + sigma eta_t.
end{align*}
The observation equation is a little bit more difficult, however:
begin{align*}
y_{t+1} = Y_{t+1} - Y_t &= (mu - frac{v_t}{2}) + sqrt{v_t}epsilon_{t+1} \
&= left(mu - frac{exp h_t}{2} right) + exp[ log sqrt{v_t}] epsilon_{t+1} \
&= left(mu - frac{exp h_t}{2}right) + expleft[ frac{h_t}{2}right] epsilon_{t+1}.
end{align*}
Why is the mean return not $0$?
stochastic-processes stochastic-calculus stochastic-integrals sde
$endgroup$
add a comment |
$begingroup$
How does the discrete time stochastic volatility model arise from the continuous time one?
I have the following continuous time stochastic volatility model. $S_t$ is the price, and $v_t$ is a variance process.
$$
dS_t = mu S_tdt + sqrt{v_t}S_t dB_{1t} \
dv_t = (theta - alpha log v_t)v_tdt + sigma v_t dB_{2t} .
$$
I'm more familiar with the discrete time version:
$$
y_t = exp(h_t/2)epsilon_t \
h_{t+1} = mu + phi(h_t - mu) + sigma_t eta_t \
h_1 sim Nleft(mu, frac{sigma^2}{1-phi^2}right).
$$
${y_t}$ are the log returns, and ${h_t}$ are the "log-volatilites." Keep in mind there might be some confusion about parameters; for example the $mu$s in each of these models are different.
How do I verify that the first discretizes into the second?
Here's my work so far. First I define $Y_t = log S_t$ and $h_t = log v_t$. Then I use Ito's lemma to get
begin{align*}
dY_t &= left(mu - frac{exp h_t}{2}right)dt + exp[h_t/2] dB_{1t}\
dh_t &= left(theta - alphalog v_t - sigma^2/2right)dt + sigma dB_{2,t}\
&= alphaleft(tilde{mu} - h_t right)dt + sigma dB_{2t}.
end{align*}
I got the state/log-vol process piece. I use the Euler method to discretize, setting $Delta t = 1$, to get
begin{align*}
h_{t+1} &= alpha tilde{mu} + h_t(1-alpha) + sigma eta_t \
&= tilde{mu}(1 - phi) + phi h_t + sigma eta_t \
&= tilde{mu} + phi(h_t - tilde{mu}) + sigma eta_t.
end{align*}
The observation equation is a little bit more difficult, however:
begin{align*}
y_{t+1} = Y_{t+1} - Y_t &= (mu - frac{v_t}{2}) + sqrt{v_t}epsilon_{t+1} \
&= left(mu - frac{exp h_t}{2} right) + exp[ log sqrt{v_t}] epsilon_{t+1} \
&= left(mu - frac{exp h_t}{2}right) + expleft[ frac{h_t}{2}right] epsilon_{t+1}.
end{align*}
Why is the mean return not $0$?
stochastic-processes stochastic-calculus stochastic-integrals sde
$endgroup$
1
$begingroup$
Be aware: crossposted.
$endgroup$
– Bob Jansen
Jul 11 '18 at 15:04
add a comment |
$begingroup$
How does the discrete time stochastic volatility model arise from the continuous time one?
I have the following continuous time stochastic volatility model. $S_t$ is the price, and $v_t$ is a variance process.
$$
dS_t = mu S_tdt + sqrt{v_t}S_t dB_{1t} \
dv_t = (theta - alpha log v_t)v_tdt + sigma v_t dB_{2t} .
$$
I'm more familiar with the discrete time version:
$$
y_t = exp(h_t/2)epsilon_t \
h_{t+1} = mu + phi(h_t - mu) + sigma_t eta_t \
h_1 sim Nleft(mu, frac{sigma^2}{1-phi^2}right).
$$
${y_t}$ are the log returns, and ${h_t}$ are the "log-volatilites." Keep in mind there might be some confusion about parameters; for example the $mu$s in each of these models are different.
How do I verify that the first discretizes into the second?
Here's my work so far. First I define $Y_t = log S_t$ and $h_t = log v_t$. Then I use Ito's lemma to get
begin{align*}
dY_t &= left(mu - frac{exp h_t}{2}right)dt + exp[h_t/2] dB_{1t}\
dh_t &= left(theta - alphalog v_t - sigma^2/2right)dt + sigma dB_{2,t}\
&= alphaleft(tilde{mu} - h_t right)dt + sigma dB_{2t}.
end{align*}
I got the state/log-vol process piece. I use the Euler method to discretize, setting $Delta t = 1$, to get
begin{align*}
h_{t+1} &= alpha tilde{mu} + h_t(1-alpha) + sigma eta_t \
&= tilde{mu}(1 - phi) + phi h_t + sigma eta_t \
&= tilde{mu} + phi(h_t - tilde{mu}) + sigma eta_t.
end{align*}
The observation equation is a little bit more difficult, however:
begin{align*}
y_{t+1} = Y_{t+1} - Y_t &= (mu - frac{v_t}{2}) + sqrt{v_t}epsilon_{t+1} \
&= left(mu - frac{exp h_t}{2} right) + exp[ log sqrt{v_t}] epsilon_{t+1} \
&= left(mu - frac{exp h_t}{2}right) + expleft[ frac{h_t}{2}right] epsilon_{t+1}.
end{align*}
Why is the mean return not $0$?
stochastic-processes stochastic-calculus stochastic-integrals sde
$endgroup$
How does the discrete time stochastic volatility model arise from the continuous time one?
I have the following continuous time stochastic volatility model. $S_t$ is the price, and $v_t$ is a variance process.
$$
dS_t = mu S_tdt + sqrt{v_t}S_t dB_{1t} \
dv_t = (theta - alpha log v_t)v_tdt + sigma v_t dB_{2t} .
$$
I'm more familiar with the discrete time version:
$$
y_t = exp(h_t/2)epsilon_t \
h_{t+1} = mu + phi(h_t - mu) + sigma_t eta_t \
h_1 sim Nleft(mu, frac{sigma^2}{1-phi^2}right).
$$
${y_t}$ are the log returns, and ${h_t}$ are the "log-volatilites." Keep in mind there might be some confusion about parameters; for example the $mu$s in each of these models are different.
How do I verify that the first discretizes into the second?
Here's my work so far. First I define $Y_t = log S_t$ and $h_t = log v_t$. Then I use Ito's lemma to get
begin{align*}
dY_t &= left(mu - frac{exp h_t}{2}right)dt + exp[h_t/2] dB_{1t}\
dh_t &= left(theta - alphalog v_t - sigma^2/2right)dt + sigma dB_{2,t}\
&= alphaleft(tilde{mu} - h_t right)dt + sigma dB_{2t}.
end{align*}
I got the state/log-vol process piece. I use the Euler method to discretize, setting $Delta t = 1$, to get
begin{align*}
h_{t+1} &= alpha tilde{mu} + h_t(1-alpha) + sigma eta_t \
&= tilde{mu}(1 - phi) + phi h_t + sigma eta_t \
&= tilde{mu} + phi(h_t - tilde{mu}) + sigma eta_t.
end{align*}
The observation equation is a little bit more difficult, however:
begin{align*}
y_{t+1} = Y_{t+1} - Y_t &= (mu - frac{v_t}{2}) + sqrt{v_t}epsilon_{t+1} \
&= left(mu - frac{exp h_t}{2} right) + exp[ log sqrt{v_t}] epsilon_{t+1} \
&= left(mu - frac{exp h_t}{2}right) + expleft[ frac{h_t}{2}right] epsilon_{t+1}.
end{align*}
Why is the mean return not $0$?
stochastic-processes stochastic-calculus stochastic-integrals sde
stochastic-processes stochastic-calculus stochastic-integrals sde
edited Jan 12 at 5:20
Taylor
asked Jul 9 '18 at 18:47
TaylorTaylor
287113
287113
1
$begingroup$
Be aware: crossposted.
$endgroup$
– Bob Jansen
Jul 11 '18 at 15:04
add a comment |
1
$begingroup$
Be aware: crossposted.
$endgroup$
– Bob Jansen
Jul 11 '18 at 15:04
1
1
$begingroup$
Be aware: crossposted.
$endgroup$
– Bob Jansen
Jul 11 '18 at 15:04
$begingroup$
Be aware: crossposted.
$endgroup$
– Bob Jansen
Jul 11 '18 at 15:04
add a comment |
1 Answer
1
active
oldest
votes
$begingroup$
I guess you can discretize the raw price process too instead of the log price process. You get
$$
S_{t+1} = S_t + mu S_t + sqrt{v_t} S_t Z_t
$$
(where $Z_t$ is a standard normal variate), or
$$
frac{S_{t+1}}{S_t} - 1 = mu + sqrt{v_t} Z_t.
$$
Got the idea from: https://arxiv.org/pdf/1707.00899.pdf
$endgroup$
add a comment |
Your Answer
StackExchange.ifUsing("editor", function () {
return StackExchange.using("mathjaxEditing", function () {
StackExchange.MarkdownEditor.creationCallbacks.add(function (editor, postfix) {
StackExchange.mathjaxEditing.prepareWmdForMathJax(editor, postfix, [["$", "$"], ["\\(","\\)"]]);
});
});
}, "mathjax-editing");
StackExchange.ready(function() {
var channelOptions = {
tags: "".split(" "),
id: "69"
};
initTagRenderer("".split(" "), "".split(" "), channelOptions);
StackExchange.using("externalEditor", function() {
// Have to fire editor after snippets, if snippets enabled
if (StackExchange.settings.snippets.snippetsEnabled) {
StackExchange.using("snippets", function() {
createEditor();
});
}
else {
createEditor();
}
});
function createEditor() {
StackExchange.prepareEditor({
heartbeatType: 'answer',
autoActivateHeartbeat: false,
convertImagesToLinks: true,
noModals: true,
showLowRepImageUploadWarning: true,
reputationToPostImages: 10,
bindNavPrevention: true,
postfix: "",
imageUploader: {
brandingHtml: "Powered by u003ca class="icon-imgur-white" href="https://imgur.com/"u003eu003c/au003e",
contentPolicyHtml: "User contributions licensed under u003ca href="https://creativecommons.org/licenses/by-sa/3.0/"u003ecc by-sa 3.0 with attribution requiredu003c/au003e u003ca href="https://stackoverflow.com/legal/content-policy"u003e(content policy)u003c/au003e",
allowUrls: true
},
noCode: true, onDemand: true,
discardSelector: ".discard-answer"
,immediatelyShowMarkdownHelp:true
});
}
});
Sign up or log in
StackExchange.ready(function () {
StackExchange.helpers.onClickDraftSave('#login-link');
});
Sign up using Google
Sign up using Facebook
Sign up using Email and Password
Post as a guest
Required, but never shown
StackExchange.ready(
function () {
StackExchange.openid.initPostLogin('.new-post-login', 'https%3a%2f%2fmath.stackexchange.com%2fquestions%2f2845825%2fdiscretizing-a-stochastic-volatility-sde%23new-answer', 'question_page');
}
);
Post as a guest
Required, but never shown
1 Answer
1
active
oldest
votes
1 Answer
1
active
oldest
votes
active
oldest
votes
active
oldest
votes
$begingroup$
I guess you can discretize the raw price process too instead of the log price process. You get
$$
S_{t+1} = S_t + mu S_t + sqrt{v_t} S_t Z_t
$$
(where $Z_t$ is a standard normal variate), or
$$
frac{S_{t+1}}{S_t} - 1 = mu + sqrt{v_t} Z_t.
$$
Got the idea from: https://arxiv.org/pdf/1707.00899.pdf
$endgroup$
add a comment |
$begingroup$
I guess you can discretize the raw price process too instead of the log price process. You get
$$
S_{t+1} = S_t + mu S_t + sqrt{v_t} S_t Z_t
$$
(where $Z_t$ is a standard normal variate), or
$$
frac{S_{t+1}}{S_t} - 1 = mu + sqrt{v_t} Z_t.
$$
Got the idea from: https://arxiv.org/pdf/1707.00899.pdf
$endgroup$
add a comment |
$begingroup$
I guess you can discretize the raw price process too instead of the log price process. You get
$$
S_{t+1} = S_t + mu S_t + sqrt{v_t} S_t Z_t
$$
(where $Z_t$ is a standard normal variate), or
$$
frac{S_{t+1}}{S_t} - 1 = mu + sqrt{v_t} Z_t.
$$
Got the idea from: https://arxiv.org/pdf/1707.00899.pdf
$endgroup$
I guess you can discretize the raw price process too instead of the log price process. You get
$$
S_{t+1} = S_t + mu S_t + sqrt{v_t} S_t Z_t
$$
(where $Z_t$ is a standard normal variate), or
$$
frac{S_{t+1}}{S_t} - 1 = mu + sqrt{v_t} Z_t.
$$
Got the idea from: https://arxiv.org/pdf/1707.00899.pdf
answered Jan 12 at 5:30
TaylorTaylor
287113
287113
add a comment |
add a comment |
Thanks for contributing an answer to Mathematics Stack Exchange!
- Please be sure to answer the question. Provide details and share your research!
But avoid …
- Asking for help, clarification, or responding to other answers.
- Making statements based on opinion; back them up with references or personal experience.
Use MathJax to format equations. MathJax reference.
To learn more, see our tips on writing great answers.
Sign up or log in
StackExchange.ready(function () {
StackExchange.helpers.onClickDraftSave('#login-link');
});
Sign up using Google
Sign up using Facebook
Sign up using Email and Password
Post as a guest
Required, but never shown
StackExchange.ready(
function () {
StackExchange.openid.initPostLogin('.new-post-login', 'https%3a%2f%2fmath.stackexchange.com%2fquestions%2f2845825%2fdiscretizing-a-stochastic-volatility-sde%23new-answer', 'question_page');
}
);
Post as a guest
Required, but never shown
Sign up or log in
StackExchange.ready(function () {
StackExchange.helpers.onClickDraftSave('#login-link');
});
Sign up using Google
Sign up using Facebook
Sign up using Email and Password
Post as a guest
Required, but never shown
Sign up or log in
StackExchange.ready(function () {
StackExchange.helpers.onClickDraftSave('#login-link');
});
Sign up using Google
Sign up using Facebook
Sign up using Email and Password
Post as a guest
Required, but never shown
Sign up or log in
StackExchange.ready(function () {
StackExchange.helpers.onClickDraftSave('#login-link');
});
Sign up using Google
Sign up using Facebook
Sign up using Email and Password
Sign up using Google
Sign up using Facebook
Sign up using Email and Password
Post as a guest
Required, but never shown
Required, but never shown
Required, but never shown
Required, but never shown
Required, but never shown
Required, but never shown
Required, but never shown
Required, but never shown
Required, but never shown
uUyYmC YHVT,K2hZAjYOiueFr 3W,5Vux5
1
$begingroup$
Be aware: crossposted.
$endgroup$
– Bob Jansen
Jul 11 '18 at 15:04