Stochastic representation formula
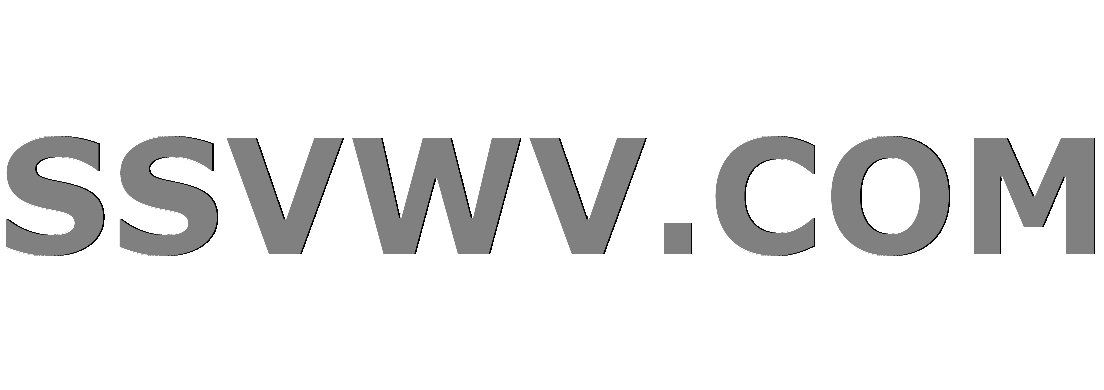
Multi tool use
$begingroup$
Consider the following boundary value problem in the domain $[0,T]$ x $R$ for an unknown function F.
$frac{partial F}{partial t}(t,x) + mu(t,x)frac{partial F}{partial x}(t,x) + frac {1}{2}sigma^2(t,x)frac{partial^2 F}{partial t^2}(t,x) = 0$
$F(T,x) = Phi(x)$
$Phi, mu, sigma$ are assumed to be known functions.
Derive a stochastic representation formula for this problem. Make sure it is clear at which points the functions should be evaluated.
So this is how I think you do this, but I need some help understanding the steps.
We first assume that it actually exists such stochastic representation that is the solution to the SDE
$dX_s = mu(t,X_s)ds + sigma(t,X_s)dB_s$
$X_t = x$
And the infinitesimal generator $mathcal{A}$ of X is
$mu(t,x)frac{partial F}{partial x}(t,x) + frac {1}{2}sigma^2(t,x)frac{partial^2 F}{partial t^2}(t,x)$
so we can rewrite the the PDE as
$frac{partial F}{partial t} + mathcal{A}F(t,x) = 0$ (or should it be a minus sign)
$F(T,x) = Phi(x)$ $(star)$
And now we apply the Itô formula on $F(s,X_s)$ and this step I don't understand (if someone could explain it I would be very pleased, I know the Itô formula but on this problem I don't get it), but if I'm correct it we get
$F(T,X_T) = F(t,X_t) + int^T_t big(frac{partial F}{partial t}(s,X_s) + mathcal{A}F(s, X_s)big)ds + int^T_tsigma(s, X_s)frac{partial F}{partial x}(s,X_s)dB_s$
(where the ds-integral is $0$ and $F(T,X_t) = Phi(X_T)$ by assumption)
So now we take expectations on both sides and we get:
$E_{t,x}[Phi(X_T)] = F(t, x) + E_{t,x}[int^T_t sigma(s,X_s)frac{partial F}{partial x}(s,X_s)dB_s]$
Where the integral is $0$ if $sigma(s,X_s)frac{partial F}{partial x}(s,X_s)$ is sufficiently nice.
So we then have our stochastic representation of $F(t,x) = E_{t,x}[Phi(X_T)]$
So, how do you apply the Itô formula on $(star)$? Also I'm a bit confused if it should be a minus sign at $(star)$ aswell, I think it should? Is this the Kolmogorovs backward equation? And if you instead have a initial condition in the PDE you get the foward equation? I think we should also be able to this if you add some nice function to the PDE, say $r(x)$. How would that change the derivation of this stochastic representation?
probability-theory stochastic-processes stochastic-integrals
$endgroup$
add a comment |
$begingroup$
Consider the following boundary value problem in the domain $[0,T]$ x $R$ for an unknown function F.
$frac{partial F}{partial t}(t,x) + mu(t,x)frac{partial F}{partial x}(t,x) + frac {1}{2}sigma^2(t,x)frac{partial^2 F}{partial t^2}(t,x) = 0$
$F(T,x) = Phi(x)$
$Phi, mu, sigma$ are assumed to be known functions.
Derive a stochastic representation formula for this problem. Make sure it is clear at which points the functions should be evaluated.
So this is how I think you do this, but I need some help understanding the steps.
We first assume that it actually exists such stochastic representation that is the solution to the SDE
$dX_s = mu(t,X_s)ds + sigma(t,X_s)dB_s$
$X_t = x$
And the infinitesimal generator $mathcal{A}$ of X is
$mu(t,x)frac{partial F}{partial x}(t,x) + frac {1}{2}sigma^2(t,x)frac{partial^2 F}{partial t^2}(t,x)$
so we can rewrite the the PDE as
$frac{partial F}{partial t} + mathcal{A}F(t,x) = 0$ (or should it be a minus sign)
$F(T,x) = Phi(x)$ $(star)$
And now we apply the Itô formula on $F(s,X_s)$ and this step I don't understand (if someone could explain it I would be very pleased, I know the Itô formula but on this problem I don't get it), but if I'm correct it we get
$F(T,X_T) = F(t,X_t) + int^T_t big(frac{partial F}{partial t}(s,X_s) + mathcal{A}F(s, X_s)big)ds + int^T_tsigma(s, X_s)frac{partial F}{partial x}(s,X_s)dB_s$
(where the ds-integral is $0$ and $F(T,X_t) = Phi(X_T)$ by assumption)
So now we take expectations on both sides and we get:
$E_{t,x}[Phi(X_T)] = F(t, x) + E_{t,x}[int^T_t sigma(s,X_s)frac{partial F}{partial x}(s,X_s)dB_s]$
Where the integral is $0$ if $sigma(s,X_s)frac{partial F}{partial x}(s,X_s)$ is sufficiently nice.
So we then have our stochastic representation of $F(t,x) = E_{t,x}[Phi(X_T)]$
So, how do you apply the Itô formula on $(star)$? Also I'm a bit confused if it should be a minus sign at $(star)$ aswell, I think it should? Is this the Kolmogorovs backward equation? And if you instead have a initial condition in the PDE you get the foward equation? I think we should also be able to this if you add some nice function to the PDE, say $r(x)$. How would that change the derivation of this stochastic representation?
probability-theory stochastic-processes stochastic-integrals
$endgroup$
$begingroup$
It would be great if someone could tell me how the Itô integral in question is solved as soon as possible, because that I really need for the exam in 2 days!!! Many thanks in advance!
$endgroup$
– Good guy Mike
Dec 10 '12 at 23:07
$begingroup$
I tried adding in the PDE so that I have: $frac{partial F}{partial t}(t,x) + mu(t,x)frac{partial F}{partial x}(t,x) + frac {1}{2}sigma^2(t,x)frac{partial^2 F}{partial t^2}(t,x) + rF(t,x) = 0$ So applying the Itô integral (which I understood now) $frac{partial F}{partial t} + mathcal{A}F(t,x) = r F(t,x)$ (instead of 0) So now I get, after taking expectation $E_{t,x}[Phi(X_T)] = F(t, x) + E_{t,x}[int^T_t rF(s,X_s)ds] + (dots) = {Fubini's} = F(t, x) + int^T_t rE_{t,x}[F(s,X_s)]ds + (dots)$ From here I'm not certain on how to continue.
$endgroup$
– Good guy Mike
Dec 11 '12 at 12:47
$begingroup$
My idea was to put $E_{t,x}[F(s,X_s)] = m(s)$ so we have that, after differentiating, $m'(t) = r m(t)$ so that means that $m(t) = e^{rt}$. But then the solution should be that $F(t,x) = e^{-rs}E_{x,t}[Phi(X_T)]$ and that is not what I get from this.
$endgroup$
– Good guy Mike
Dec 11 '12 at 12:52
add a comment |
$begingroup$
Consider the following boundary value problem in the domain $[0,T]$ x $R$ for an unknown function F.
$frac{partial F}{partial t}(t,x) + mu(t,x)frac{partial F}{partial x}(t,x) + frac {1}{2}sigma^2(t,x)frac{partial^2 F}{partial t^2}(t,x) = 0$
$F(T,x) = Phi(x)$
$Phi, mu, sigma$ are assumed to be known functions.
Derive a stochastic representation formula for this problem. Make sure it is clear at which points the functions should be evaluated.
So this is how I think you do this, but I need some help understanding the steps.
We first assume that it actually exists such stochastic representation that is the solution to the SDE
$dX_s = mu(t,X_s)ds + sigma(t,X_s)dB_s$
$X_t = x$
And the infinitesimal generator $mathcal{A}$ of X is
$mu(t,x)frac{partial F}{partial x}(t,x) + frac {1}{2}sigma^2(t,x)frac{partial^2 F}{partial t^2}(t,x)$
so we can rewrite the the PDE as
$frac{partial F}{partial t} + mathcal{A}F(t,x) = 0$ (or should it be a minus sign)
$F(T,x) = Phi(x)$ $(star)$
And now we apply the Itô formula on $F(s,X_s)$ and this step I don't understand (if someone could explain it I would be very pleased, I know the Itô formula but on this problem I don't get it), but if I'm correct it we get
$F(T,X_T) = F(t,X_t) + int^T_t big(frac{partial F}{partial t}(s,X_s) + mathcal{A}F(s, X_s)big)ds + int^T_tsigma(s, X_s)frac{partial F}{partial x}(s,X_s)dB_s$
(where the ds-integral is $0$ and $F(T,X_t) = Phi(X_T)$ by assumption)
So now we take expectations on both sides and we get:
$E_{t,x}[Phi(X_T)] = F(t, x) + E_{t,x}[int^T_t sigma(s,X_s)frac{partial F}{partial x}(s,X_s)dB_s]$
Where the integral is $0$ if $sigma(s,X_s)frac{partial F}{partial x}(s,X_s)$ is sufficiently nice.
So we then have our stochastic representation of $F(t,x) = E_{t,x}[Phi(X_T)]$
So, how do you apply the Itô formula on $(star)$? Also I'm a bit confused if it should be a minus sign at $(star)$ aswell, I think it should? Is this the Kolmogorovs backward equation? And if you instead have a initial condition in the PDE you get the foward equation? I think we should also be able to this if you add some nice function to the PDE, say $r(x)$. How would that change the derivation of this stochastic representation?
probability-theory stochastic-processes stochastic-integrals
$endgroup$
Consider the following boundary value problem in the domain $[0,T]$ x $R$ for an unknown function F.
$frac{partial F}{partial t}(t,x) + mu(t,x)frac{partial F}{partial x}(t,x) + frac {1}{2}sigma^2(t,x)frac{partial^2 F}{partial t^2}(t,x) = 0$
$F(T,x) = Phi(x)$
$Phi, mu, sigma$ are assumed to be known functions.
Derive a stochastic representation formula for this problem. Make sure it is clear at which points the functions should be evaluated.
So this is how I think you do this, but I need some help understanding the steps.
We first assume that it actually exists such stochastic representation that is the solution to the SDE
$dX_s = mu(t,X_s)ds + sigma(t,X_s)dB_s$
$X_t = x$
And the infinitesimal generator $mathcal{A}$ of X is
$mu(t,x)frac{partial F}{partial x}(t,x) + frac {1}{2}sigma^2(t,x)frac{partial^2 F}{partial t^2}(t,x)$
so we can rewrite the the PDE as
$frac{partial F}{partial t} + mathcal{A}F(t,x) = 0$ (or should it be a minus sign)
$F(T,x) = Phi(x)$ $(star)$
And now we apply the Itô formula on $F(s,X_s)$ and this step I don't understand (if someone could explain it I would be very pleased, I know the Itô formula but on this problem I don't get it), but if I'm correct it we get
$F(T,X_T) = F(t,X_t) + int^T_t big(frac{partial F}{partial t}(s,X_s) + mathcal{A}F(s, X_s)big)ds + int^T_tsigma(s, X_s)frac{partial F}{partial x}(s,X_s)dB_s$
(where the ds-integral is $0$ and $F(T,X_t) = Phi(X_T)$ by assumption)
So now we take expectations on both sides and we get:
$E_{t,x}[Phi(X_T)] = F(t, x) + E_{t,x}[int^T_t sigma(s,X_s)frac{partial F}{partial x}(s,X_s)dB_s]$
Where the integral is $0$ if $sigma(s,X_s)frac{partial F}{partial x}(s,X_s)$ is sufficiently nice.
So we then have our stochastic representation of $F(t,x) = E_{t,x}[Phi(X_T)]$
So, how do you apply the Itô formula on $(star)$? Also I'm a bit confused if it should be a minus sign at $(star)$ aswell, I think it should? Is this the Kolmogorovs backward equation? And if you instead have a initial condition in the PDE you get the foward equation? I think we should also be able to this if you add some nice function to the PDE, say $r(x)$. How would that change the derivation of this stochastic representation?
probability-theory stochastic-processes stochastic-integrals
probability-theory stochastic-processes stochastic-integrals
edited Dec 11 '12 at 11:05
Good guy Mike
asked Dec 10 '12 at 23:04
Good guy MikeGood guy Mike
435
435
$begingroup$
It would be great if someone could tell me how the Itô integral in question is solved as soon as possible, because that I really need for the exam in 2 days!!! Many thanks in advance!
$endgroup$
– Good guy Mike
Dec 10 '12 at 23:07
$begingroup$
I tried adding in the PDE so that I have: $frac{partial F}{partial t}(t,x) + mu(t,x)frac{partial F}{partial x}(t,x) + frac {1}{2}sigma^2(t,x)frac{partial^2 F}{partial t^2}(t,x) + rF(t,x) = 0$ So applying the Itô integral (which I understood now) $frac{partial F}{partial t} + mathcal{A}F(t,x) = r F(t,x)$ (instead of 0) So now I get, after taking expectation $E_{t,x}[Phi(X_T)] = F(t, x) + E_{t,x}[int^T_t rF(s,X_s)ds] + (dots) = {Fubini's} = F(t, x) + int^T_t rE_{t,x}[F(s,X_s)]ds + (dots)$ From here I'm not certain on how to continue.
$endgroup$
– Good guy Mike
Dec 11 '12 at 12:47
$begingroup$
My idea was to put $E_{t,x}[F(s,X_s)] = m(s)$ so we have that, after differentiating, $m'(t) = r m(t)$ so that means that $m(t) = e^{rt}$. But then the solution should be that $F(t,x) = e^{-rs}E_{x,t}[Phi(X_T)]$ and that is not what I get from this.
$endgroup$
– Good guy Mike
Dec 11 '12 at 12:52
add a comment |
$begingroup$
It would be great if someone could tell me how the Itô integral in question is solved as soon as possible, because that I really need for the exam in 2 days!!! Many thanks in advance!
$endgroup$
– Good guy Mike
Dec 10 '12 at 23:07
$begingroup$
I tried adding in the PDE so that I have: $frac{partial F}{partial t}(t,x) + mu(t,x)frac{partial F}{partial x}(t,x) + frac {1}{2}sigma^2(t,x)frac{partial^2 F}{partial t^2}(t,x) + rF(t,x) = 0$ So applying the Itô integral (which I understood now) $frac{partial F}{partial t} + mathcal{A}F(t,x) = r F(t,x)$ (instead of 0) So now I get, after taking expectation $E_{t,x}[Phi(X_T)] = F(t, x) + E_{t,x}[int^T_t rF(s,X_s)ds] + (dots) = {Fubini's} = F(t, x) + int^T_t rE_{t,x}[F(s,X_s)]ds + (dots)$ From here I'm not certain on how to continue.
$endgroup$
– Good guy Mike
Dec 11 '12 at 12:47
$begingroup$
My idea was to put $E_{t,x}[F(s,X_s)] = m(s)$ so we have that, after differentiating, $m'(t) = r m(t)$ so that means that $m(t) = e^{rt}$. But then the solution should be that $F(t,x) = e^{-rs}E_{x,t}[Phi(X_T)]$ and that is not what I get from this.
$endgroup$
– Good guy Mike
Dec 11 '12 at 12:52
$begingroup$
It would be great if someone could tell me how the Itô integral in question is solved as soon as possible, because that I really need for the exam in 2 days!!! Many thanks in advance!
$endgroup$
– Good guy Mike
Dec 10 '12 at 23:07
$begingroup$
It would be great if someone could tell me how the Itô integral in question is solved as soon as possible, because that I really need for the exam in 2 days!!! Many thanks in advance!
$endgroup$
– Good guy Mike
Dec 10 '12 at 23:07
$begingroup$
I tried adding in the PDE so that I have: $frac{partial F}{partial t}(t,x) + mu(t,x)frac{partial F}{partial x}(t,x) + frac {1}{2}sigma^2(t,x)frac{partial^2 F}{partial t^2}(t,x) + rF(t,x) = 0$ So applying the Itô integral (which I understood now) $frac{partial F}{partial t} + mathcal{A}F(t,x) = r F(t,x)$ (instead of 0) So now I get, after taking expectation $E_{t,x}[Phi(X_T)] = F(t, x) + E_{t,x}[int^T_t rF(s,X_s)ds] + (dots) = {Fubini's} = F(t, x) + int^T_t rE_{t,x}[F(s,X_s)]ds + (dots)$ From here I'm not certain on how to continue.
$endgroup$
– Good guy Mike
Dec 11 '12 at 12:47
$begingroup$
I tried adding in the PDE so that I have: $frac{partial F}{partial t}(t,x) + mu(t,x)frac{partial F}{partial x}(t,x) + frac {1}{2}sigma^2(t,x)frac{partial^2 F}{partial t^2}(t,x) + rF(t,x) = 0$ So applying the Itô integral (which I understood now) $frac{partial F}{partial t} + mathcal{A}F(t,x) = r F(t,x)$ (instead of 0) So now I get, after taking expectation $E_{t,x}[Phi(X_T)] = F(t, x) + E_{t,x}[int^T_t rF(s,X_s)ds] + (dots) = {Fubini's} = F(t, x) + int^T_t rE_{t,x}[F(s,X_s)]ds + (dots)$ From here I'm not certain on how to continue.
$endgroup$
– Good guy Mike
Dec 11 '12 at 12:47
$begingroup$
My idea was to put $E_{t,x}[F(s,X_s)] = m(s)$ so we have that, after differentiating, $m'(t) = r m(t)$ so that means that $m(t) = e^{rt}$. But then the solution should be that $F(t,x) = e^{-rs}E_{x,t}[Phi(X_T)]$ and that is not what I get from this.
$endgroup$
– Good guy Mike
Dec 11 '12 at 12:52
$begingroup$
My idea was to put $E_{t,x}[F(s,X_s)] = m(s)$ so we have that, after differentiating, $m'(t) = r m(t)$ so that means that $m(t) = e^{rt}$. But then the solution should be that $F(t,x) = e^{-rs}E_{x,t}[Phi(X_T)]$ and that is not what I get from this.
$endgroup$
– Good guy Mike
Dec 11 '12 at 12:52
add a comment |
1 Answer
1
active
oldest
votes
$begingroup$
I use the notation $frac{partial}{partial t}F = F_t$.
$dF = F_t,dt + F_x,dX_t + F_{xx}frac{1}{2}sigma,dt$
Assume that your original PDE is equal to $rF$ instead of 0 (set $r$ to 0 to get the original PDE).
Put $Z(s) = e^{-int_t^s r,du}F(s, X(s))$. Then
$dZ(s) = -re^{-int_t^s r,du}Fds + e^{-int_t^s r,du}dF = e^{-int_t^s r,du}(dF - rFds)$
So, $Z(T) - Z(t) = int_t^T dZ(s)$ and
$Z(T) = Z(t) + int_t^T e^{-int_t^s r,du}(F_t + F_xmu + F_{xx}frac{1}{2}sigma -rF),ds + int_t^T e^{-int_t^s r,du}F_xsigma,dW(s)$.
The time integral vanishes. Then, since the expectation of the stochastic integral is zero, the expected value of $Z(T)$ is:
$E_t[Z(T)] = E_t[Z(t)] = e^{-int_t^t r,ds}F(t, X(t)) = F(t, x)$.
On the other hand, $Z(T)$ is defined as $e^{-int_t^T r,ds}F(T, X(T)) = e^{-int_t^T r,ds}Phi(X(T))$
so we conclude:
$E_t[e^{-int_t^T r,ds}Phi(X(T))] = F(t, x)$.
$endgroup$
add a comment |
Your Answer
StackExchange.ifUsing("editor", function () {
return StackExchange.using("mathjaxEditing", function () {
StackExchange.MarkdownEditor.creationCallbacks.add(function (editor, postfix) {
StackExchange.mathjaxEditing.prepareWmdForMathJax(editor, postfix, [["$", "$"], ["\\(","\\)"]]);
});
});
}, "mathjax-editing");
StackExchange.ready(function() {
var channelOptions = {
tags: "".split(" "),
id: "69"
};
initTagRenderer("".split(" "), "".split(" "), channelOptions);
StackExchange.using("externalEditor", function() {
// Have to fire editor after snippets, if snippets enabled
if (StackExchange.settings.snippets.snippetsEnabled) {
StackExchange.using("snippets", function() {
createEditor();
});
}
else {
createEditor();
}
});
function createEditor() {
StackExchange.prepareEditor({
heartbeatType: 'answer',
autoActivateHeartbeat: false,
convertImagesToLinks: true,
noModals: true,
showLowRepImageUploadWarning: true,
reputationToPostImages: 10,
bindNavPrevention: true,
postfix: "",
imageUploader: {
brandingHtml: "Powered by u003ca class="icon-imgur-white" href="https://imgur.com/"u003eu003c/au003e",
contentPolicyHtml: "User contributions licensed under u003ca href="https://creativecommons.org/licenses/by-sa/3.0/"u003ecc by-sa 3.0 with attribution requiredu003c/au003e u003ca href="https://stackoverflow.com/legal/content-policy"u003e(content policy)u003c/au003e",
allowUrls: true
},
noCode: true, onDemand: true,
discardSelector: ".discard-answer"
,immediatelyShowMarkdownHelp:true
});
}
});
Sign up or log in
StackExchange.ready(function () {
StackExchange.helpers.onClickDraftSave('#login-link');
});
Sign up using Google
Sign up using Facebook
Sign up using Email and Password
Post as a guest
Required, but never shown
StackExchange.ready(
function () {
StackExchange.openid.initPostLogin('.new-post-login', 'https%3a%2f%2fmath.stackexchange.com%2fquestions%2f255852%2fstochastic-representation-formula%23new-answer', 'question_page');
}
);
Post as a guest
Required, but never shown
1 Answer
1
active
oldest
votes
1 Answer
1
active
oldest
votes
active
oldest
votes
active
oldest
votes
$begingroup$
I use the notation $frac{partial}{partial t}F = F_t$.
$dF = F_t,dt + F_x,dX_t + F_{xx}frac{1}{2}sigma,dt$
Assume that your original PDE is equal to $rF$ instead of 0 (set $r$ to 0 to get the original PDE).
Put $Z(s) = e^{-int_t^s r,du}F(s, X(s))$. Then
$dZ(s) = -re^{-int_t^s r,du}Fds + e^{-int_t^s r,du}dF = e^{-int_t^s r,du}(dF - rFds)$
So, $Z(T) - Z(t) = int_t^T dZ(s)$ and
$Z(T) = Z(t) + int_t^T e^{-int_t^s r,du}(F_t + F_xmu + F_{xx}frac{1}{2}sigma -rF),ds + int_t^T e^{-int_t^s r,du}F_xsigma,dW(s)$.
The time integral vanishes. Then, since the expectation of the stochastic integral is zero, the expected value of $Z(T)$ is:
$E_t[Z(T)] = E_t[Z(t)] = e^{-int_t^t r,ds}F(t, X(t)) = F(t, x)$.
On the other hand, $Z(T)$ is defined as $e^{-int_t^T r,ds}F(T, X(T)) = e^{-int_t^T r,ds}Phi(X(T))$
so we conclude:
$E_t[e^{-int_t^T r,ds}Phi(X(T))] = F(t, x)$.
$endgroup$
add a comment |
$begingroup$
I use the notation $frac{partial}{partial t}F = F_t$.
$dF = F_t,dt + F_x,dX_t + F_{xx}frac{1}{2}sigma,dt$
Assume that your original PDE is equal to $rF$ instead of 0 (set $r$ to 0 to get the original PDE).
Put $Z(s) = e^{-int_t^s r,du}F(s, X(s))$. Then
$dZ(s) = -re^{-int_t^s r,du}Fds + e^{-int_t^s r,du}dF = e^{-int_t^s r,du}(dF - rFds)$
So, $Z(T) - Z(t) = int_t^T dZ(s)$ and
$Z(T) = Z(t) + int_t^T e^{-int_t^s r,du}(F_t + F_xmu + F_{xx}frac{1}{2}sigma -rF),ds + int_t^T e^{-int_t^s r,du}F_xsigma,dW(s)$.
The time integral vanishes. Then, since the expectation of the stochastic integral is zero, the expected value of $Z(T)$ is:
$E_t[Z(T)] = E_t[Z(t)] = e^{-int_t^t r,ds}F(t, X(t)) = F(t, x)$.
On the other hand, $Z(T)$ is defined as $e^{-int_t^T r,ds}F(T, X(T)) = e^{-int_t^T r,ds}Phi(X(T))$
so we conclude:
$E_t[e^{-int_t^T r,ds}Phi(X(T))] = F(t, x)$.
$endgroup$
add a comment |
$begingroup$
I use the notation $frac{partial}{partial t}F = F_t$.
$dF = F_t,dt + F_x,dX_t + F_{xx}frac{1}{2}sigma,dt$
Assume that your original PDE is equal to $rF$ instead of 0 (set $r$ to 0 to get the original PDE).
Put $Z(s) = e^{-int_t^s r,du}F(s, X(s))$. Then
$dZ(s) = -re^{-int_t^s r,du}Fds + e^{-int_t^s r,du}dF = e^{-int_t^s r,du}(dF - rFds)$
So, $Z(T) - Z(t) = int_t^T dZ(s)$ and
$Z(T) = Z(t) + int_t^T e^{-int_t^s r,du}(F_t + F_xmu + F_{xx}frac{1}{2}sigma -rF),ds + int_t^T e^{-int_t^s r,du}F_xsigma,dW(s)$.
The time integral vanishes. Then, since the expectation of the stochastic integral is zero, the expected value of $Z(T)$ is:
$E_t[Z(T)] = E_t[Z(t)] = e^{-int_t^t r,ds}F(t, X(t)) = F(t, x)$.
On the other hand, $Z(T)$ is defined as $e^{-int_t^T r,ds}F(T, X(T)) = e^{-int_t^T r,ds}Phi(X(T))$
so we conclude:
$E_t[e^{-int_t^T r,ds}Phi(X(T))] = F(t, x)$.
$endgroup$
I use the notation $frac{partial}{partial t}F = F_t$.
$dF = F_t,dt + F_x,dX_t + F_{xx}frac{1}{2}sigma,dt$
Assume that your original PDE is equal to $rF$ instead of 0 (set $r$ to 0 to get the original PDE).
Put $Z(s) = e^{-int_t^s r,du}F(s, X(s))$. Then
$dZ(s) = -re^{-int_t^s r,du}Fds + e^{-int_t^s r,du}dF = e^{-int_t^s r,du}(dF - rFds)$
So, $Z(T) - Z(t) = int_t^T dZ(s)$ and
$Z(T) = Z(t) + int_t^T e^{-int_t^s r,du}(F_t + F_xmu + F_{xx}frac{1}{2}sigma -rF),ds + int_t^T e^{-int_t^s r,du}F_xsigma,dW(s)$.
The time integral vanishes. Then, since the expectation of the stochastic integral is zero, the expected value of $Z(T)$ is:
$E_t[Z(T)] = E_t[Z(t)] = e^{-int_t^t r,ds}F(t, X(t)) = F(t, x)$.
On the other hand, $Z(T)$ is defined as $e^{-int_t^T r,ds}F(T, X(T)) = e^{-int_t^T r,ds}Phi(X(T))$
so we conclude:
$E_t[e^{-int_t^T r,ds}Phi(X(T))] = F(t, x)$.
edited Jan 7 at 9:12
answered Jan 17 '14 at 21:59
HunaphuHunaphu
1256
1256
add a comment |
add a comment |
Thanks for contributing an answer to Mathematics Stack Exchange!
- Please be sure to answer the question. Provide details and share your research!
But avoid …
- Asking for help, clarification, or responding to other answers.
- Making statements based on opinion; back them up with references or personal experience.
Use MathJax to format equations. MathJax reference.
To learn more, see our tips on writing great answers.
Sign up or log in
StackExchange.ready(function () {
StackExchange.helpers.onClickDraftSave('#login-link');
});
Sign up using Google
Sign up using Facebook
Sign up using Email and Password
Post as a guest
Required, but never shown
StackExchange.ready(
function () {
StackExchange.openid.initPostLogin('.new-post-login', 'https%3a%2f%2fmath.stackexchange.com%2fquestions%2f255852%2fstochastic-representation-formula%23new-answer', 'question_page');
}
);
Post as a guest
Required, but never shown
Sign up or log in
StackExchange.ready(function () {
StackExchange.helpers.onClickDraftSave('#login-link');
});
Sign up using Google
Sign up using Facebook
Sign up using Email and Password
Post as a guest
Required, but never shown
Sign up or log in
StackExchange.ready(function () {
StackExchange.helpers.onClickDraftSave('#login-link');
});
Sign up using Google
Sign up using Facebook
Sign up using Email and Password
Post as a guest
Required, but never shown
Sign up or log in
StackExchange.ready(function () {
StackExchange.helpers.onClickDraftSave('#login-link');
});
Sign up using Google
Sign up using Facebook
Sign up using Email and Password
Sign up using Google
Sign up using Facebook
Sign up using Email and Password
Post as a guest
Required, but never shown
Required, but never shown
Required, but never shown
Required, but never shown
Required, but never shown
Required, but never shown
Required, but never shown
Required, but never shown
Required, but never shown
2Il42vAm2cEggA,5LxlUiAmNX8Rr7en8Bi79bd,eo12xN19rQYGhrs65g1,LbYwmoW2mvugQv1WpFPaOY,RFx2eKd,n
$begingroup$
It would be great if someone could tell me how the Itô integral in question is solved as soon as possible, because that I really need for the exam in 2 days!!! Many thanks in advance!
$endgroup$
– Good guy Mike
Dec 10 '12 at 23:07
$begingroup$
I tried adding in the PDE so that I have: $frac{partial F}{partial t}(t,x) + mu(t,x)frac{partial F}{partial x}(t,x) + frac {1}{2}sigma^2(t,x)frac{partial^2 F}{partial t^2}(t,x) + rF(t,x) = 0$ So applying the Itô integral (which I understood now) $frac{partial F}{partial t} + mathcal{A}F(t,x) = r F(t,x)$ (instead of 0) So now I get, after taking expectation $E_{t,x}[Phi(X_T)] = F(t, x) + E_{t,x}[int^T_t rF(s,X_s)ds] + (dots) = {Fubini's} = F(t, x) + int^T_t rE_{t,x}[F(s,X_s)]ds + (dots)$ From here I'm not certain on how to continue.
$endgroup$
– Good guy Mike
Dec 11 '12 at 12:47
$begingroup$
My idea was to put $E_{t,x}[F(s,X_s)] = m(s)$ so we have that, after differentiating, $m'(t) = r m(t)$ so that means that $m(t) = e^{rt}$. But then the solution should be that $F(t,x) = e^{-rs}E_{x,t}[Phi(X_T)]$ and that is not what I get from this.
$endgroup$
– Good guy Mike
Dec 11 '12 at 12:52