Pros and cons of multivariate interpolation techniques for scattered data?
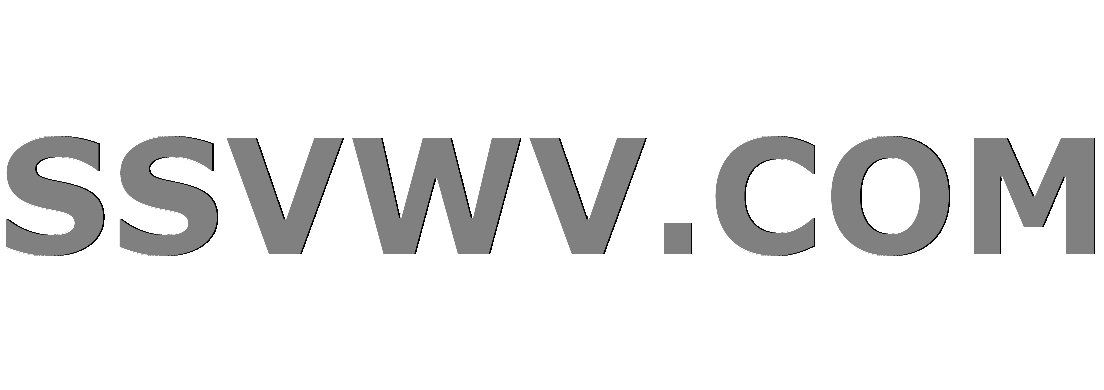
Multi tool use
$begingroup$
I have a numerical simulation $f$ that takes 6 input parameters $mathbf x = x_1, x_2, ldots x_6$. I have randomly selected $25,000$ random combinations of these inputs and calculated $f(mathbf x)$. The output of the simulation is about 25 numbers $mathbf y = y_1, y_2, ldots y_{25}$ (although for the sake of simplicity we could pretend I am only computing $y_1$).
I am now trying to interpolate this function 6-dimensional function. I hold out a model, train on the remaining models, and test on the held-out model.
I have tried various schemes:
Linear interpolation (scipy.interpolate.griddata)
Artificial neural network (sklearn.neural_network.MLPRegressor)
Kriging / Gaussian Process Regression (sklearn.gaussian_process.gaussianProcessRegressor)
Polynomial ridge regression (e.g., polynomial features)
Distance-weighted nearest neighbors (K Nearest Neighbors)
To my surprise, linear interpolation has outperformed the rest. (To be clear, I have tried various data preprocessing steps to ensure the data are normalized, etc., as well as several hours changing the parameters of each method.)
Is this what I should have expected? Is there another technique that may work better?
I would like to note that I have apparently failed to find a method that can do cubic interpolation with high dimensional input, which I would imagine to be a promising route. (SciPy at least is limited to 2D functions.)
numerical-methods regression interpolation simulation
$endgroup$
add a comment |
$begingroup$
I have a numerical simulation $f$ that takes 6 input parameters $mathbf x = x_1, x_2, ldots x_6$. I have randomly selected $25,000$ random combinations of these inputs and calculated $f(mathbf x)$. The output of the simulation is about 25 numbers $mathbf y = y_1, y_2, ldots y_{25}$ (although for the sake of simplicity we could pretend I am only computing $y_1$).
I am now trying to interpolate this function 6-dimensional function. I hold out a model, train on the remaining models, and test on the held-out model.
I have tried various schemes:
Linear interpolation (scipy.interpolate.griddata)
Artificial neural network (sklearn.neural_network.MLPRegressor)
Kriging / Gaussian Process Regression (sklearn.gaussian_process.gaussianProcessRegressor)
Polynomial ridge regression (e.g., polynomial features)
Distance-weighted nearest neighbors (K Nearest Neighbors)
To my surprise, linear interpolation has outperformed the rest. (To be clear, I have tried various data preprocessing steps to ensure the data are normalized, etc., as well as several hours changing the parameters of each method.)
Is this what I should have expected? Is there another technique that may work better?
I would like to note that I have apparently failed to find a method that can do cubic interpolation with high dimensional input, which I would imagine to be a promising route. (SciPy at least is limited to 2D functions.)
numerical-methods regression interpolation simulation
$endgroup$
add a comment |
$begingroup$
I have a numerical simulation $f$ that takes 6 input parameters $mathbf x = x_1, x_2, ldots x_6$. I have randomly selected $25,000$ random combinations of these inputs and calculated $f(mathbf x)$. The output of the simulation is about 25 numbers $mathbf y = y_1, y_2, ldots y_{25}$ (although for the sake of simplicity we could pretend I am only computing $y_1$).
I am now trying to interpolate this function 6-dimensional function. I hold out a model, train on the remaining models, and test on the held-out model.
I have tried various schemes:
Linear interpolation (scipy.interpolate.griddata)
Artificial neural network (sklearn.neural_network.MLPRegressor)
Kriging / Gaussian Process Regression (sklearn.gaussian_process.gaussianProcessRegressor)
Polynomial ridge regression (e.g., polynomial features)
Distance-weighted nearest neighbors (K Nearest Neighbors)
To my surprise, linear interpolation has outperformed the rest. (To be clear, I have tried various data preprocessing steps to ensure the data are normalized, etc., as well as several hours changing the parameters of each method.)
Is this what I should have expected? Is there another technique that may work better?
I would like to note that I have apparently failed to find a method that can do cubic interpolation with high dimensional input, which I would imagine to be a promising route. (SciPy at least is limited to 2D functions.)
numerical-methods regression interpolation simulation
$endgroup$
I have a numerical simulation $f$ that takes 6 input parameters $mathbf x = x_1, x_2, ldots x_6$. I have randomly selected $25,000$ random combinations of these inputs and calculated $f(mathbf x)$. The output of the simulation is about 25 numbers $mathbf y = y_1, y_2, ldots y_{25}$ (although for the sake of simplicity we could pretend I am only computing $y_1$).
I am now trying to interpolate this function 6-dimensional function. I hold out a model, train on the remaining models, and test on the held-out model.
I have tried various schemes:
Linear interpolation (scipy.interpolate.griddata)
Artificial neural network (sklearn.neural_network.MLPRegressor)
Kriging / Gaussian Process Regression (sklearn.gaussian_process.gaussianProcessRegressor)
Polynomial ridge regression (e.g., polynomial features)
Distance-weighted nearest neighbors (K Nearest Neighbors)
To my surprise, linear interpolation has outperformed the rest. (To be clear, I have tried various data preprocessing steps to ensure the data are normalized, etc., as well as several hours changing the parameters of each method.)
Is this what I should have expected? Is there another technique that may work better?
I would like to note that I have apparently failed to find a method that can do cubic interpolation with high dimensional input, which I would imagine to be a promising route. (SciPy at least is limited to 2D functions.)
numerical-methods regression interpolation simulation
numerical-methods regression interpolation simulation
asked Dec 16 '18 at 12:14


rhombidodecahedronrhombidodecahedron
6281619
6281619
add a comment |
add a comment |
0
active
oldest
votes
Your Answer
StackExchange.ifUsing("editor", function () {
return StackExchange.using("mathjaxEditing", function () {
StackExchange.MarkdownEditor.creationCallbacks.add(function (editor, postfix) {
StackExchange.mathjaxEditing.prepareWmdForMathJax(editor, postfix, [["$", "$"], ["\\(","\\)"]]);
});
});
}, "mathjax-editing");
StackExchange.ready(function() {
var channelOptions = {
tags: "".split(" "),
id: "69"
};
initTagRenderer("".split(" "), "".split(" "), channelOptions);
StackExchange.using("externalEditor", function() {
// Have to fire editor after snippets, if snippets enabled
if (StackExchange.settings.snippets.snippetsEnabled) {
StackExchange.using("snippets", function() {
createEditor();
});
}
else {
createEditor();
}
});
function createEditor() {
StackExchange.prepareEditor({
heartbeatType: 'answer',
autoActivateHeartbeat: false,
convertImagesToLinks: true,
noModals: true,
showLowRepImageUploadWarning: true,
reputationToPostImages: 10,
bindNavPrevention: true,
postfix: "",
imageUploader: {
brandingHtml: "Powered by u003ca class="icon-imgur-white" href="https://imgur.com/"u003eu003c/au003e",
contentPolicyHtml: "User contributions licensed under u003ca href="https://creativecommons.org/licenses/by-sa/3.0/"u003ecc by-sa 3.0 with attribution requiredu003c/au003e u003ca href="https://stackoverflow.com/legal/content-policy"u003e(content policy)u003c/au003e",
allowUrls: true
},
noCode: true, onDemand: true,
discardSelector: ".discard-answer"
,immediatelyShowMarkdownHelp:true
});
}
});
Sign up or log in
StackExchange.ready(function () {
StackExchange.helpers.onClickDraftSave('#login-link');
});
Sign up using Google
Sign up using Facebook
Sign up using Email and Password
Post as a guest
Required, but never shown
StackExchange.ready(
function () {
StackExchange.openid.initPostLogin('.new-post-login', 'https%3a%2f%2fmath.stackexchange.com%2fquestions%2f3042545%2fpros-and-cons-of-multivariate-interpolation-techniques-for-scattered-data%23new-answer', 'question_page');
}
);
Post as a guest
Required, but never shown
0
active
oldest
votes
0
active
oldest
votes
active
oldest
votes
active
oldest
votes
Thanks for contributing an answer to Mathematics Stack Exchange!
- Please be sure to answer the question. Provide details and share your research!
But avoid …
- Asking for help, clarification, or responding to other answers.
- Making statements based on opinion; back them up with references or personal experience.
Use MathJax to format equations. MathJax reference.
To learn more, see our tips on writing great answers.
Sign up or log in
StackExchange.ready(function () {
StackExchange.helpers.onClickDraftSave('#login-link');
});
Sign up using Google
Sign up using Facebook
Sign up using Email and Password
Post as a guest
Required, but never shown
StackExchange.ready(
function () {
StackExchange.openid.initPostLogin('.new-post-login', 'https%3a%2f%2fmath.stackexchange.com%2fquestions%2f3042545%2fpros-and-cons-of-multivariate-interpolation-techniques-for-scattered-data%23new-answer', 'question_page');
}
);
Post as a guest
Required, but never shown
Sign up or log in
StackExchange.ready(function () {
StackExchange.helpers.onClickDraftSave('#login-link');
});
Sign up using Google
Sign up using Facebook
Sign up using Email and Password
Post as a guest
Required, but never shown
Sign up or log in
StackExchange.ready(function () {
StackExchange.helpers.onClickDraftSave('#login-link');
});
Sign up using Google
Sign up using Facebook
Sign up using Email and Password
Post as a guest
Required, but never shown
Sign up or log in
StackExchange.ready(function () {
StackExchange.helpers.onClickDraftSave('#login-link');
});
Sign up using Google
Sign up using Facebook
Sign up using Email and Password
Sign up using Google
Sign up using Facebook
Sign up using Email and Password
Post as a guest
Required, but never shown
Required, but never shown
Required, but never shown
Required, but never shown
Required, but never shown
Required, but never shown
Required, but never shown
Required, but never shown
Required, but never shown
Vn9nbiLt,yXI6Bz,a6egxn,wlb UttkO4u 8T f,9nHcMS,37UdlvM BDUkKDcHMm,M9NKHBw